We’re hosing down the hype surrounding AI, and frankly, we’re going to need a bigger hose.
Large language models like ChatGPT have landed in a big way, and we’re still figuring out what this all means. The risks are real, but it’s less about avoiding hostile robot takeovers and more about fighting misinformation and creating a data ecology that benefits everyone. How do we protect creators and counter some of the bad ideologies surrounding AI? It’s a big chat with Dr Emily Bender of the University of Washington, cohost of the podcast Mystery AI Hype Theatre 3000.
Also: how do computers do on humour? Surprisingly well! We’re talking to Dr Jack Hessel, co-author of some new award-winning research that uses New Yorker cartoons. Is humour the last frontier for computational linguistics? Do computers “understand” humour? What makes something funny?
Listen to this episode
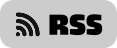

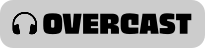
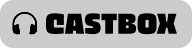

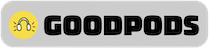

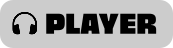

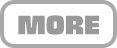
Video promo
Patreon supporters
Huge thanks to all our great patrons! Your support means a lot to us. Special thanks to:
- Iztin
- Termy
- Elías
- Matt
- Whitney
- Helen
- Jack
- PharaohKatt
- LordMortis
- gramaryen
- Larry
- Kristofer
- Andy
- James
- Nigel
- Meredith
- Kate
- Nasrin
- Joanna
- Keith
- Ayesha
- Steele
- Margareth
- Manú
- Rodger
- Rhian
- Colleen
- Ignacio
- Sonic Snejhog
- Kevin
- Jeff
- Andy from Logophilius
- Stan
- Kathy
- Rach
- Cheyenne
- Felicity
- Amir
- Canny Archer
- O Tim
- Alyssa
- Chris
- Laurie
- aengry balls
- Tadhg
- and bumping up to the Supporter level, ✨Nikoli✨
And our newest patrons:
At the Listener level:
- Ned
- Sam
- Holly, Spider Hunter of Taipei
And at the Friend level: Mitch
Become a Patreon supporter yourself and get access to bonus episodes and more!
Become a Patron!Show notes
Ryan Reynolds announces launch of ‘Welsh Wednesdays’ on his new US TV channel
https://nation.cymru/news/ryan-reynolds-announces-launch-of-welsh-wednesdays-on-his-new-us-tv-channel/
Maximum Effort | Wikipedia
https://en.wikipedia.org/wiki/Maximum_Effort
Ukraine: Refugee children almost fluent in Welsh
https://www.bbc.com/news/uk-wales-66179694
Boring Report
https://www.boringreport.org/app
Not lost in translation: The implications of machine translation technologies for language professionals and for broader society
https://www.oecd-ilibrary.org/social-issues-migration-health/not-lost-in-translation_e1d1d170-en
Discrepancies in autobiographical memories— implications for the assessment of asylum seekers: repeated interviews study
https://www.ncbi.nlm.nih.gov/pmc/articles/PMC65293/
How NOT to use the Ethnologue (Wayback Machine copy)
https://web.archive.org/web/20221122214247/https://www.ethnologue.com/ethnoblog/m-paul-lewis/how-not-use-ethnologue#.Y31Cby3P1fA
Do Androids Laugh at Electric Sheep? Humor “Understanding” Benchmarks from The New Yorker Caption Contest
https://arxiv.org/abs/2209.06293
Best Papers | ACL 2023
https://2023.aclweb.org/program/best_papers/
Allen Institute for AI
https://allenai.org
jmhessel/caption_contest_corpus | github
https://github.com/jmhessel/caption_contest_corpus
jmhessel/newyorker_caption_contest | Hugging Face
https://huggingface.co/datasets/jmhessel/newyorker_caption_contest
Humor Can Be Dissected, as a Frog Can, But the Thing Dies in the Process
https://quoteinvestigator.com/2014/10/14/frog/
Automating Inequality: How High-Tech Tools Profile, Police, and Punish the Poor, by Virginia Eubanks | Macmillan Publishers
https://us.macmillan.com/books/9781250074317/automatinginequality
What is Automation Bias? | Databricks
https://www.databricks.com/glossary/automation-bias
The #BenderRule: On Naming the Languages We Study and Why It Matters
https://thegradient.pub/the-benderrule-on-naming-the-languages-we-study-and-why-it-matters/
Chirag Shah, Emily M. Bender: Situating Search
https://dl.acm.org/doi/10.1145/3498366.3505816
All-knowing machines are a fantasy
https://iai.tv/articles/all-knowing-machines-are-a-fantasy-auid-2334
Research Philosophy | DAIR (Distributed AI Research Project)
https://www.dair-institute.org/research/
[PDF] Combating Harmful Hype in Natural Language Processing
https://pml4dc.github.io/iclr2023/pdf/PML4DC_ICLR2023_39.pdf
Gavin Abercrombie et al.: Mirages: On Anthropomorphism in Dialogue Systems
https://arxiv.org/abs/2305.09800
Former TikTok Moderator Threatens Lawsuit in Kenya Over Alleged Trauma and Unfair Dismissal by Billy Perigo | TIME Magazine
https://time.com/6293271/tiktok-bytedance-kenya-moderator-lawsuit/
Potentially Useful, but Error-Prone: ChatGPT on the Black Tech Ecosystem
https://tpinsights.com/potentially-useful-but-error-prone-chatgpt-on-the-black-tech-ecosystem/
Rich Felker, @dalias@hachyderm.io
AI is a lot like fossil fuel industry. Seizing and burning something (in this case, the internet, and more broadly, written-down human knowledge) that was built up over a long time much faster than it could ever be replenished.
https://hachyderm.io/@dalias/110528154854288688
Understanding TESCREAL with Dr. Timnit Gebru and Émile Torres
https://pod.co/dave-troy/understanding-tescreal-with-dr-timnit-gebru-and-mile-torres
Keep your AI claims in check
https://www.ftc.gov/business-guidance/blog/2023/02/keep-your-ai-claims-check
Chatbots, deepfakes, and voice clones: AI deception for sale
https://www.ftc.gov/business-guidance/blog/2023/03/chatbots-deepfakes-voice-clones-ai-deception-sale
The Luring Test: AI and the engineering of consumer trust
https://www.ftc.gov/business-guidance/blog/2023/05/luring-test-ai-engineering-consumer-trust
Deciphering the Recent FTC Trilogy of Blog Posts on Artificial Intelligence
https://www.jdsupra.com/legalnews/deciphering-the-recent-ftc-trilogy-of-1214436/
‘We’ll Never Undermine the Privacy Promise of People Who Rely on Us’: Signal’s Meredith Whittaker | The Wire
https://thewire.in/tech/ai-privacy-tech-signal-president-meredith-whittaker-interview
The Artificial Intelligence Act
https://artificialintelligenceact.eu
Sasha Costanza-Chock: Design Justice, A.I., and Escape from the Matrix of Domination
https://cmsw.mit.edu/design-justice-ai-escape-matrix-of-domination/
Bender & Koller (2020). Climbing towards NLU: On Meaning, Form, and Understanding in the Age of Data. 🐙
https://www.aclweb.org/anthology/2020.acl-main.463/
Can machines learn how to behave? by Blaise Aguera y Arcas
https://medium.com/@blaisea/can-machines-learn-how-to-behave-42a02a57fadb
Mystery AI Hype Theater 3000
https://peertube.dair-institute.org/c/mystery_ai_hype_theater/videos?s=1
What Is Kanekalon? The Viral TikTok Song Might Not Be For You
https://stylecaster.com/beauty/hair-nails/1609166/what-is-kanekalon/
Kaneka Corporation | Wikipedia
https://en.wikipedia.org/wiki/Kaneka_Corporation
From delulu to rizz, 2023’s most viral internet slang defined
https://mashable.com/article/internet-dictionary-2023
What is ‘Rat Girl Summer’? A breakdown of TikTok’s latest and very attainable self-care attitude for 2023
https://www.myimperfectlife.com/features/what-is-rat-girl-summer-tiktok
Fake Eli Lilly Twitter Account Claims Insulin Is Free, Stock Falls 4.37%
https://www.forbes.com/sites/brucelee/2022/11/12/fake-eli-lilly-twitter-account-claims-insulin-is-free-stock-falls-43/?sh=40ab42bb41a3
Twitter Blue accounts fuel Ukraine War misinformation
https://www.bbc.com/news/world-europe-66113460
Twitter Blue Accounts Are Spreading Misinformation About The War In Ukraine
https://www.forbes.com/sites/petersuciu/2023/07/10/twitter-blue-accounts-are-spreading-misinformation-about-the-war-in-ukraine/
Far-right Twitter influencers first on Elon Musk’s monetization scheme
https://www.washingtonpost.com/technology/2023/07/13/twitter-creators-payments-right-wing/
Twitter Starts Paying People to Tweet, Far-Right Accounts Among First Payouts
https://au.pcmag.com/social-media/100814/twitter-starts-paying-people-to-tweet-far-right-accounts-among-first-payouts
Why Some People Dump Their Traumas on Us
https://www.psychologytoday.com/au/blog/spellbound/202111/why-some-people-dump-their-traumas-us
Transcript
[Transcript provided by SpeechDocs Podcast Transcription]
DANIEL: I’m here with Emily Dr… [LAUGHS] Emily Dr Bender.
EMILY: [LAUGHS]
DANIEL: This is why we get a take two.
EMILY: Right.
[BECAUSE LANGUAGE THEME]
DANIEL: Hello and welcome to Because Language. A show about linguistics, the science of language. My name is Daniel Midgley. Let’s meet the team. It’s everyone’s favourite linguist, except in Perth, Australia, where I am everyone’s favourite linguist. It’s Hedvig Skirgård.
HEDVIG: Um, thank you, Daniel. Yes, it’s me, Hedvig. I… um… Fuck. You always do this.
BEN: [LAUGHS]
DANIEL: You can be the favourite linguist everywhere else, just not…
HEDVIG: No… I don’t want to be the favourite linguist because then I have to, like, behave, and I can’t be, like, mischievous…
BEN: She’s… yeah, no, no… Hedvig’s far too Scandi to be everyone’s favourite linguist because she wants to be able to be blunt and to the point. And you can’t be that if you’re, like, making everyone happy all the time.
HEDVIG: And I want to make people… sometimes, I think it’s good to be a bit uncomfortable. And I want to have the liberty to make people uncomfortable.
DANIEL: No matter what you say, I’m just going to say: That’s part of your appeal.
HEDVIG: Fuck.
[LAUGHTER]
HEDVIG: I hate this. Okay. Yeah, anyway, next one. I’m not alone as a cohost on this show. Please do the next one, please.
DANIEL: It’s everyone’s favourite human in some weirdly specific category that I was going to invent but didn’t have time to. It’s Ben Ainslie.
BEN: Okay. I now pass the chalice to Hedvig. Hedvig, what weirdly specific category am I people’s favourite human of?
DANIEL: Ben is everyone’s favourite…
HEDVIG: Biking media teacher at a sort of religious-y school.
BEN: Okay, there we go. I’ll take it. That is hyperspecific, and I’m willing to say I’m probably people’s favourite bicycle-using media teacher at a semi-religious school. There we go.
DANIEL: Okay, great. Yeah.
BEN: I can just feel somewhere else in, like, Australia right now there’s like one other guy who’s just like: I’ve been challenged.
[LAUGHTER]
DANIEL: “You guys suck.” Well, thank you both for being here. It’s great to see you. Let’s talk about this episode. Lots of stuff going on.
BEN: We’re talking about Ben Ainslie’s favourite, favourite thing to ramble on: AI.
DANIEL: Mm-hmm.
HEDVIG: Mm-hmm.
DANIEL: We’ve got a lot of AI coming here. Our special guest for this episode is Dr Emily Bender of the University of Washington…
HEDVIG: Woop woop.
DANIEL: …and star of the new podcast, Mystery AI Hype Theater 3000. That’s her new podcast. Check it out.
HEDVIG: That’s such a good name and she’s so cool.
BEN: That is an excellent title.
DANIEL: She is cool.
HEDVIG: She’s very cool. She’s maybe too cool for us.
BEN: I like when people slum it with us. I really appreciate that. So, good on her for lowering herself to our level. I appreciate that.
DANIEL: Having hung out with Dr Bender in her office — because I was there in the USA, and I said, “Dr Bender, can I come over to your office and we can record a thing?” — so, having done that, I can say she is way too cool for us.
HEDVIG: What’s her office like?
BEN: You intangibly interacted with the coolness. You’ve touched the coolness and you’re like: Yep, too cool.
DANIEL: A little bit stuck to me. It’s true. Emily Bender is famous for originating the Bender Rule, which is… anyone?
BEN: I really want to make a Futurama joke, but I’m not going to.
DANIEL: No, it’s not.
HEDVIG: The Bender Rule is that when you do something about language and lots of languages, especially in the NLP space, you should mention what language you use that’s input to your model. And this rule exists because most of the time, it is English, but it’s never mentioned. So, they just say, “Our new model performs really well at predicting blah, blah, blah in language!” And it’s like, “What language? What did you do?” And you have to like…
BEN: There’s 4,000 of the fucking things! Just name one!
HEDVIG: “Yeah, just say which one it is, please!”
DANIEL: Mhm! The Bender Rule has become formulated as: Always name the language you’re working on. We’ve had Dr Bender as a guest on the show back in the Talk the Talk days in 2018. That was Episode 335, 100 Things. But since that time, Emily has been relentless in talking about the problems inherent in AI, when we make the mistake of… um… this is not to put words in her mouth, but I’ve noticed that for Dr Bender, it’s very important that AI gets used in a way that is just, inclusive, and equitable for everyone. And what we’re seeing is that things are not getting set up in that kind of way. There are some real dangers ahead and some fake dangers. And we talked about which one is which. And also, there’s an octopus, so that’s fun.
HEDVIG: Oh.
BEN: Well, that’s a juicy tidbit to drop at the end! You have successfully whet my appetite, sir.
DANIEL: We’re also talking to Dr Jack Hessel of the Allen Institute for AI. He’s worked on a paper that won an award for Best Paper at the recent ACL Conference. That’s the Association for Computational Linguistics. The paper was about humour, and it used data from New Yorker cartoons. How is that not good?
HEDVIG: That’s really cool! That’s really cool. And best paper, that’s got to be a really good paper. Also, from the Allen Institute?
DANIEL: That’s correct.
HEDVIG: AI?
DANIEL: I know. AI for AI.
HEDVIG: That is funny. That’s good.
DANIEL: Our next episode is a Mailbag episode, and we still might have room for a good question. You can hear that episode the minute it comes out if you’re a patron at the Listener level. But no matter your level, you’ll be helping to keep the show going. All patrons get to join us on live episodes and all patrons get to hang out with us on our Discord server.
HEDVIG: They’re really nice.
BEN: And we’ve got a hangout coming up with them in the not-too-distant future, which genuinely on my sad little life is like a proper, actual, like, “I look forward to this thing” thing.
DANIEL: [LAUGHS] Yep!
HEDVIG: Yeah. Of course, it is.
BEN: Definitely.
DANIEL: We have so many…
HEDVIG: Also, I suck at planning. When is it?
[LAUGHTER]
DANIEL: Keep your eyes on your Patreon account just for all the details because I’ll put them up there when they’re happening. We have so many great patrons who have been with us from the very beginning of Because Language and even many from the Talk the Talk era. But we do lose some patrons every month through the normal process of attrition. Sometimes their interests change, sometimes their financial situation changes. So, of course, we’re trying to keep as many patrons as we can and attract new ones. If you’d like to join us and our great linguistic community, why don’t you just wander over to patreon.com/becauselangpod. We’ll make you glad you did.
BEN: It’s pretty great being one of our patrons.
DANIEL: Well, I think so.
BEN: Um, so, can we go on to the next thing now?
DANIEL: Yep.
HEDVIG: [GIGGLES]
BEN: I’m just checking.
HEDVIG: Okay. Just take a deep breath.
BEN: He’s a man who likes to do a lot of plugs. Now, some of you might look out there at the world in the news ecosystem — the newsosystem — and see nothing but a fetid swamp with putrefaction and methane fires and just general awfulness all the time. But like with a real swamp, if you take just a little sample of that and you put it under a microscope and you see this wonderful world come to life of all sorts of different things. That’s what we like to do here in our news section, is we take just little tiny bits here and there that are linguistically flavoured, and we bring them to you and show you what is interesting about the hellscape of the swamp. So, Daniel, what stories from the swamp do we have today?
DANIEL: I’m just impressed that you managed to innovate the neologism NEWSOSYSTEM. That was good. That was smooth.
BEN: Yup. What can I say, Daniel, 12 years and I can do word… mingling.
DANIEL: This one comes to us from Cheyenne on our Discord server. It’s about Welsh. And it’s about the actor, Ryan Reynolds.
HEDVIG: He…? Ryan Reynolds?
BEN: He’s not Welsh, is he?
HEDVIG: No, he’s not. He’s American as far as I know.
BEN: He’s Canadian.
DANIEL: He’s Canadian.
HEDVIG: Canadian, okay. He’s North American. Oh, god, I mistook a Canadian, I told them they were American a couple of weeks ago and I still cringe when I think about it.
BEN: It’s not a nice thing to call a non-American.
HEDVIG: I’m very very s… No, it’s not. They really hate it. But I need a term that is like… okay, never mind. Anyway, Ryan Reynolds, as far as I know, has been interested in UK football, hasn’t he?
DANIEL: Yeah!
BEN: He bought a football team alongside the guy from It’s Always Sunny in Philadelphia. Yes.
HEDVIG: Yes.
DANIEL: Yes, it was Rob McElhenney. Am I saying his name right?
BEN: McElhenney?
HEDVIG: Is this related to that? Has he bought a Welsh team?
DANIEL: Yes, the team that they bought is Wrexham. It’s a town in Northeastern Wales. But it’s not just about football. They’re also opening up… with their production company, Maximum Effort, they’re producing Welsh programs for US television.
BEN: Oh!
HEDVIG: Oh, really?
DANIEL: He says, “As many have noted, there is an alarming lack of Welsh content available for American viewing pleasure. That stops today. Well, actually, Wednesdays. We’re so grateful to S4C” — that’s a Welsh language broadcasting channel — “for helping bring Welsh programming to a broader audience, and to that broader audience, don’t worry, I am told there will be subtitles.” It’s going to be six hours every Wednesday in Welsh.
BEN: Six hours of Welsh language programming on American TV, presumably on cable, I’m guessing.
DANIEL: Yes. A lot of it’s going to be… I forget the channel that it’s going to be on, but it’s going to be there. How about that?
HEDVIG: That’s really fun. I like this. Ryan Reynolds clearly, like, has some personal interest, and he is funding… He’s like, “I like UK football, I’m going to buy a, like, mid-tier team.” And I don’t know, it’s like…
BEN: Yeah, they’ve done a whole show around it, from what understand. Right? Like, mI am on record as being one of the least sportsball-focused males ever to grace the face of the Earth, but even I, when I’m scrolling through Disney+, can see the Wrexham United, like, TV show, which is like the reality TV show that follows this thing. And here’s the other thing, right…
HEDVIG: Is it just wholesome?
BEN: It is wholesome, but what he’s doing, you kind of have to do. Right? Like, can you imagine the opposite of this? So, a guy with his movie-star friend, both of whom are North American, buy a Welsh team and then proceed to give no fucks or interest about Welshness or Welshdom or any…. They’d be eaten alive.
DANIEL: Bad look. Bad look.
BEN: The Welsh would cook them over an open fire.
DANIEL: Is that what they do?
HEDVIG: But he had a choice. He could have bought an English team and they probably would not have been as like… they wouldn’t be like, “Yoh, you need to promote English language.” They probably would have been like: We’re good.
BEN: Do we have any idea whether either of them have Welsh heritage at least?
DANIEL: Not that I know of; they picked the team just for team-related reasons.
HEDVIG: I don’t think so.
BEN: ‘Cause it was goin’ cheap! They looked at the list price and they were like, “That one’s affordable.” I’m with Hedvig. This is inherently a very cool thing because minority languages should always get more play. It’s unfortunate, obviously, that with all other things, there’s a sort of proximity of privilege type thing going on here. Which is like, Welsh being white and Western and Eurocentric as a minority language is going to get far more attention and funding than, say, I don’t know, Noongar or some other Indigenous language from the Canada or the US or something. Which doesn’t mean Welsh shouldn’t get funding and stuff, it’s a minority language and it’s great that we’re uplifting it and celebrating it. But it’s also worth acknowledging that, yeah, this is always how it plays out. The stuff closest to a privileged position will get attention first and radiate outward from there.
DANIEL: Suppose so. It’s a good candidate. I mean, it’s got a lot of fans. There are a lot of people who love Welsh. So, you know, they’re leveraging that love and using their privilege to do something good.
BEN: It’s a good template as well hopefully, right?
DANIEL: Yeah.
BEN: It’d be great if people can look at this and be like, “Wow.” The Welsh revival process can be like a playbook that other minority languages try and draw from as, like a… what I can see as a layperson, a really successful… right? Like, I assume Welsh was nearly extinct in the same way nearly all the other minority languages were at a certain point, and everyone went, “Fuck! We’ve got to do something.”
HEDVIG: It also helps that they want to distance themselves from England more, and then…
[LAUGHTER]
HEDVIG: …if you want to form a nation, you’re like, “What do we have that they don’t have? We have this.” Um, a couple of years ago, I went to Cardiff to see friend of the show Seán Roberts, and went to an all-Welsh club where they played music. And he said that over 10 years ago, a lot of the songs that were produced in Welsh were… the lyrics were about Welsh being endangered and Welsh culture being under threat. And now, the lyrics are about, like: “I love you, you love me.”
BEN: Yeah. “We are going to get turnt!” But in Welsh.
DANIEL: [LAUGHS]
HEDVIG: Yeah. And I took that to mean it’s broadened, it’s a more inclusive thing. Speaking of Welsh revival right now, I also saw in the news, which I think Daniel did as well, about the Ukrainian refugees in Wales. Did you guys read this?
DANIEL: Yeah. Why don’t you give us that story?
BEN: What a great segue.
HEDVIG: Yeah, no. So I saw BBC News were reporting that Ukrainian refugees who have settled in Wales and are going to Welsh schools, there are some Welsh schools where they have immersion programs for learning Welsh. So, the kiddos are learning Welsh. And they’re also learning English alongside it. So, everyone who’s freaking out on Twitter can calm themselves. Because there are a lot of English people being like, “Why are they only learning Welsh? Why are they also learning English?” They’re fine. They’re going to be fine. They’re going to pick up English.
DANIEL: They’re fine.
HEDVIG: So now we have little trilingual, Ukrainian, Welsh, English kiddos. That’s pretty cute.
BEN: What a delightfully… like the linguistic version of Boggle, like, you press the thing and it just goes [MAKES BOGGLE SOUNDS]
[LAUGHTER]
DANIEL: Well, we hope we can see positive things coming out of that. Okay, Ben.
BEN: Yes.
DANIEL: A couple of episodes… oh, it was in our Fake News episode with Jack Grieve that news should be boring.
BEN: Oh, about how it’s boring? Yeah. Here, let me just really clearly state Ben Ainslie’s patented based-on-nothing-at-all-other-than-the-thoughts-that-are-in-my-head belief.
DANIEL: Yes, please.
BEN: Good news is boring. The metaphor that I always bring up is: it’s eating your vegetables, which is actually a really shitty thing to say because I really like to cook and I know how to make vegetables super tasty and delicious and awesome. But if we think back to, like, awful, boil-everything English cooking, good news is like eating your vegetables, or at least it should be.
DANIEL: Okay.
HEDVIG: And by that good news, you mean news that have a high quality, not news about positive events.
BEN: Yes, yes, sorry. News that fulfills the fourth estate function well is boring.
DANIEL: Well, it turns out that there’s a new app in town, the Boring Report.
BEN: [LAUGHS] I think they just call that the ABC, Daniel.
DANIEL: [LAUGHS] No, this isn’t the ABC. So, it’s an app that uses the power of AI to turn sensationalist news into regular boring news.
BEN: Oh!
HEDVIG: Yesssssss.
BEN: That’s good.
DANIEL: So, here’s the tagline, “By utilising the power of enhanced AI language models capable of generating humanlike text, Boring Report processes exciting news articles and transforms them into the content that you see. This helps readers focus on the essential details and minimises the impact of sensationalism.”
HEDVIG: That’s really cool.
BEN: That is a neat idea. I like that.
DANIEL: I don’t know how it works. I don’t know how they’ve decided what sensationalism looks like, but…
BEN: Yeah, yeah. There’s a lot of black boxing going on here, isn’t there?
DANIEL: There is, but you can look at the Boring Report article, and then you can also just pull up a little tab there and see what the original article looks like.
BEN: Oh, you can unboring it.
DANIEL: That’s right. So, what I’m going to do here is I’m just looking up an article about climate change, and this one comes from NBC News. Let me just find it. The title of this article is Heat, Flooding, and Smoke: The US is in the midst of a summer of extremes, and this is by Evan Bush. “The word of the summer is ‘extreme.’ Extreme flooding, extreme heat, extreme smoke. Scientists have predicted a climate of extremes in report after report as the Earth warms because humans continue to belch fossil fuel pollution into the atmosphere. And now, it’s here, with a dizzying slew of broken records and heartbreaking scenes.” That was the NBC article.
BEN: Okay, I will admit that I found that really gross and heavy-handed. [LAUGHS]
DANIEL: Really strong, right? Strong author’s voice. Here’s the Boring Report’s version. “Climate change and summer weather. This summer has seen a rise in extreme weather events, including flooding, heat, and smoke. These occurrences are consistent with predictions made by scientists regarding global warming due to human activities.”
HEDVIG: Cool.
BEN: Yeah, okay.
DANIEL: I like the boring part. That was all right.
BEN: It’s tricky. Like I said… I’m now going to contradict myself because I’m a wanker. I said that good news has to be boring, but I also do concede that a certain amount of connectivity, connection, what’s the word I’m looking for here?
HEDVIG: [SINGS TAUNTINGLY] Ben is changing his mind.
BEN: Not changing my mind but…
HEDVIG: [SINGS MORE TAUNTINGLY] Ben is a flip-flopper.
[LAUGHTER]
BEN: Get off the fence, Ainslie. I will concede that certain language needs to be used to get people to care. Right?
DANIEL: Yeah. There’s a purpose for a mode of language.
HEDVIG: This is a full reversal. This is a full reversal, and you know it!
BEN: No, oh…
DANIEL: No, I thought of this too, actually.
HEDVIG: Because it was about news that you thought were important and that you thought people should eat their vegetables. So, you wanted to say, “Maybe we do need to put extra salt on it. Maybe they do get to put ketchup on their vegetables.” Which I agree with.
BEN: No no no. Okay. Let’s continue that analogy. Okay. I like that. I like that because I think I do believe that we should put seasonings on vegetables. But what I don’t believe is that instead of vegetables, we should give people just, like, horrendously processed, like…
DANIEL: Cheese sticks.
BEN: Yeah, right? Or like fish fingers or whatever. Right?
DANIEL: Yeah. So, this is a very interesting use of summarisation. If you like it, check it out. It’s the Boring Report. And again, what I like about it is you can read the boring version and then you can read the real version and you can give feedback on whether you thought it was good or not. So, if you like boring news, that’s the place to go.
This one was suggested to us by Elizabeth via Patreon. “Hi, team. I thought you might like this new report from the OECD on machine translation.” The OECD, [MAKES HALTING SOUNDS] I should know what this stands for.
HEDVIG: OECD countries, it’s an organisation of countries with high GDP and high industrialisation. It stands for…
BEN: How have all three of us forgotten this?
HEDVIG: OECD. We can google.
DANIEL: It’s the Organisation for Economic Cooperation and Development. Okay. Elizabeth says, “Following on from the great ChatGPT episode earlier this year, the article is called Not lost in translation: The implications of machine translation technologies for language professionals and for broader society. It’s by Francesca Borgonovi, Justine Hervé, and Helke Seitz. Here are the takeaways that I got when I was reading through it. And I’m just going to read some pulls here.
“Machine translation technologies can play a key role to facilitate interlanguage communication. Machine translation, in fact, can deliver output cheaply, timely(ly), and quickly and can tackle large volumes of data to be translated. However, the quality of the output remains lower than the quality of the output delivered by professional language professionals, especially for translations from and to low resource languages and text loaded with cultural significance.”
I’m going to skip down. “Analyses based on data on online job vacancies for language professionals for a selected number of OECD countries between 2014 and 2019 indicate that the introduction of higher quality machine translation systems did not lead to decreases in the demand for language professionals.” And finally, “In high stakes settings, machine translation tools remain inadequate and the use of language professionals or even teams of language professionals should be privileged.” Those are my main takeaways.
BEN: I think that’s a really important thing to finish on as well. I think it’s really easy — and I’m guilty of this — when we talk about translating and stuff, I think people imagine someone being a UN ambassador’s translator and doing cool work on the floor of the UN. But we’ve got to remember that, like, in family court, a person will need to translate in a family violence situation, or I notice in the article they talk about in warfare situations. Like in Afghanistan, a translator’s job can mean literally the difference of life and death for many different people, potentially. So, it’s just… I’m really glad that they acknowledge [CHUCKLES] this stuff literally has the power to kill if used incorrectly or improperly, and it’s worth remembering.
HEDVIG: And they also point out, which we’ve seen before, is that when people seek asylum, if they first get interviewed and they tell one story and they later get interviewed and there’s any discrepancy, even if that is very minimal… in this case, it was whether an event was carried out solo or multiple people, and the machine translation had mistakenly translated a singular pronoun into a plural one. So, the judge was like, “You said you were alone doing this, but this paper says there was more than one of you.” And it’s like… Even if that actually doesn’t carry so much weight in the overall story — maybe you were two people running instead of one — the way a lot of asylum courts work in a lot of countries, including the US and in Sweden, and I’m sure Australia, if you find one hole in the asylum seeker’s story, that’s enough legally to say that they can’t be trusted at all and you can dismiss the entire case.
DANIEL: Yep.
HEDVIG: And this happens with these kind of things, and if there’s not support on hand, if there’s not other experts who can weigh in and say, “Look, we’ve had a second look at this and this doesn’t make sense,” you can get dismissed. There was a case a couple of years ago where the US immigration was using the Ethnologue SILs catalog of languages to determine whether a refugee’s story was true because they said, “Oh, I speak this language and I live in this country.” And they were like, “Ethnologue doesn’t register that language for that country,” because it’s like a border situation and the rest of the country is on the other side of the border. And Ethnologue had to issue an official statement saying, like, “Please, we are not a definitive…!
BEN: Under no circumstances should you…! [LAUGHS]
HEDVIG: Like, “Please don’t use us this way!” And I think a lot of people don’t realise… like, if you find one thing that’s different or not reliable in the story, however trivial, it’s like, “Oh, you said you weren’t carrying many bags, but then later you said you were very tired from carrying a lot of things,” they’re like, “Ah-ha! Liar! Argh.” They’re so strict about it.
DANIEL: Yeah. We need to emphasise this. Overreliance on machine translation in high stake settings is very, very bad. We already saw on our last episode, Forensic Linguistics, Really, with Helen Fraser, Georgina Heydon, and Diana Eades. Even a human interpreter is not some kind of neutral conductor of meaning, like a tube or some kind of window. And a machine translator is also not neutral, even though we might be fooled into thinking it is because math, right? I think that’s going to be the big message of our show today. AI is not neutral, and we need to remember that. Thanks, Elizabeth, for that story. You can check that out. There’s a link on our website becauselanguage.com.
Let’s finish the news with the chat with Dr Jack Hessel. He’s been working on a paper which won an award for best paper at the latest ACL Conference, the Association for Computational Linguistics. This is about detecting humor in New Yorker cartoons. I love New Yorker cartoons. They are the best.
BEN: I personally think Larson would have been a better contender. That’s just me.
DANIEL: Why are people still attached to Gary Larson?
BEN: Oh, I think he’s infinitely superior to New Yorker as a body of work.
DANIEL: Oh, really?
BEN: Yeah. Big time.
DANIEL: Okay.
BEN: I find New York cartoons insipid of the highest order.
DANIEL: [LAUGHS] Oh, wow.
BEN: One of my friends was literally given like a 600-page book of New Yorker cartoons as a gift.
DANIEL: I have that book!
BEN: And I immediately was like, “Who fucking hates you? Why would anyone give this to you?” I’m so sorry. Sorry, Garfield and New York cartoons are the same level for me.
DANIEL: Oh, my god. Okay.
HEDVIG: I don’t consume much of either, and I don’t think I know what INSIPID means.
DANIEL: Flat and silly.
BEN: Yeah, just, like…
DANIEL: Bland.
BEN: I don’t know. A dead fish is an insipid thing.
DANIEL: Ah, no, I love New Yorker cartoons. I do. And if you don’t like New Yorker cartoons, there’s a contest where you can suggest your own captions for the panels.
BEN: Oh, see, that I can see as being really fun, because the crowd always does better than the individual, right?
DANIEL: Yeah. Well, what Dr Hessel and the team did was, they decided to see if the system could pick the winners.
HEDVIG: Ohh, that’s smart! That’s smart.
BEN: Okay, let’s do it. That’s a fun idea. So, as much as I find New Yorker’s insipid, the core concept of this story is really interesting. And I’m on board.
HEDVIG: Mm-hmm.
DANIEL: I’m here with Dr Jack Hessel of the Allen Institute for AI and co-author on a paper. It won a best paper award at ACL 2023. And the paper is called Do Androids Laugh at Electric Sheep? humour “Understanding” Benchmarks from The New Yorker Caption Contest. Jack, thanks for coming on the show, talking to me today.
JACK HESSEL: Well, thanks so much for having me, Daniel.
DANIEL: “Understanding”. You’ve got the word understanding, humour “understanding” in quotes, scare quotes. I think I understand why, but what was the thinking behind that?
JACK: I think in the field of AI, there is some question, almost a philosophical question, about whether or not machines can understand anything. So, what does it mean for humans to understand something? What does it mean for machines to understand something? I think the common way in AI that people challenge machines with understanding tasks is to make little multiple-choice tests or little evaluations that have some objective score at the end. But even if they do well at these tasks, you’re sort of left with this conundrum at the end where: is this machine doing well at some multiple-choice task, and is it really understanding if it’s a machine? Is understanding intrinsic to humans? And so, in an attempt to circumvent this philosophical discussion, we decided that putting it in the quotes was maybe a good way to go.
DANIEL: Ahh, piking out. Okay, fine.
JACK: Taking a neutral approach.
[CHUCKLES]
DANIEL: Mm-hmm. No, I get that. And then you could go the other way, and you could say, “The computer is not understanding us, but do we understand each other?” You know, this kind of thing.
JACK: Exactly. About once a year I go down a philosophical rabbit hole for about a week or two, and it could be a [DANIEL LAUGHS] fun time, but it’s also not necessarily the most, on paper, productive time for me.
DANIEL: You’re walking through waves of people just thinking, “These are all stochastic parrots.”
JACK: [LAUGHS] Yes, indeed. And then, I wonder sometimes, “Do I think they’re stochastic parrots?” I think this is all above my pay grade. I’m just a computer scientist.
DANIEL: Okay, well, we’re just going to try to float above that and maybe get to some of those along the way. So, your medium for this paper was New Yorker cartoons. Do you like New Yorker cartoons, or are you someone who hates fun?
JACK: [LAUGHS] I like this dichotomy. I do like them. I often feel I’m not smart enough to understand them. I’ve looked at so many now as a result of this work, and I hate to say, but I’m not sure my rate of understanding the joke has gone up at all. If anything, maybe it’s gone down.
DANIEL: Oh, that’s interesting. Okay, so wait a minute. You’re a human, you have experience…
JACK: Allegedly.
DANIEL: …with… [LAUGHS] I’m pretty sure that you’re a human. You’ve got human experience; what’s blocking you from… If you don’t understand a certain New Yorker cartoon — one of these one-panel deals with a caption — what’s getting in your way of understanding that cartoon as a human?
JACK: Yeah, so there’s a ton that goes into New Yorker cartoons and captions. I should say that the dataset we were looking at was just from the caption contest ones. And the New Yorker has been publishing non-caption contest cartoons for nearly its 100-year history at this point. So, I have most experience with just sort of a narrow slice of even the cartoons that The New Yorker publishes.
But talking about understanding the caption contest ones, there’s a lot that goes into them. I mean, it’s not all encompassing in terms of the humor it embodies. So, it’s from a very narrow slice of humor. It’s from a very narrow culture of humor. There’s many New York jokes that I simply don’t get as someone who does not live in New York. And honestly, sometimes I have no reason to not get it other than that I don’t make the connections.
So, I can often have these jokes explained to me later, and I can sort of look back post hoc and say, “Okay, I get it,” but yeah, maybe I’m just not sharp enough all the time to get all of them.
DANIEL: Okay, so one of the things that I’ve always been drawn to about computer humor and we’ve done shows about this, we looked at a project that did, “That’s what she said” jokes. It was able to see when to put them in. But this is very sophisticated. And I’ve often thought that humour is one of those things that it will be the last frontier of natural language processing because there’s so much about humour that is human and that specifically is not computery. Like, humans have real-world knowledge about things, humans have social knowledge, and humans have an almost intuitive understanding of what counts as context and what doesn’t. And when we’re understanding a joke or we have a joke before us, we’re immediately able to select, usually, the things that count and the things that don’t count. And those aspects of our combined social knowledge that are known to be common and those that aren’t. And I feel like computers are just bad at that. Is humour sort of the last frontier?
JACK: Yeah, that’s a great point. So, prior to this project, I think I felt similarly to you, where I thought for sure there’s no way models would be able to do this. Yeah, there’s a lot of real-world knowledge that is required. A lot of humor is about violating norms. And to understand if something is a norm violation, presumably one would have to quote-unquote “understand” that something is a norm to begin with. By the way, maybe I should preface, every time I say “understanding,” you can imagine me doing scare quotes or something like that.
DANIEL: I will imagine you air quoting. Very good. Yes, “understanding.”
JACK: That’s a vain effort to circumvent this.
DANIEL: I’ll do it too. Computers are “understanding.” Okay, good, I got it.
JACK: [LAUGHS] Yeah. So, I can mention one example that I like, which ended up being our figure one. So, sort of our main one we highlight on the first page of things. It’s a cartoon by Drew Dernavich. It’s a bunch of, I guess, businesspeople sitting around a table, and they all have giant mugs of tea and coffee. And The New Yorker-selected finalist… I forget if it was the winner, but the finalist was, “Can you please pass the cow?” And this is something that requires… there’s no literal overlap between the image and the caption. Like, we’re not naming any object that’s actually depicted in the image. But yeah, there’s multi-hop reasoning of understanding that… okay, the mugs are very big and that we usually put cream or milk in tea or in coffee, and to put a lot of cream and milk into something, you might need a whole cow’s worth. This multi-hop process, I would have thought would have been quite difficult for machines. And I think in some sense it is still difficult, like we have a bunch of experiments and along none of the axes we probe for do machines match human performance. But they still do better than I thought they would.
One of our tasks is matching a caption to one of the cartoons, relative to some distractors. So, we randomly pull some captions from other contests. So different cartoons, and we say, “Okay, computer, which of these is the correct caption for this particular cartoon?” And they do better than I thought, still a gap, but they’re able to make connections about real-world knowledge, like social norms. They’re able to invoke real-world named entities. They’re able to do a lot of the skills that I find myself doing when I’m trying to understand one of these things. Not to say that they’re doing the same thing that I’m doing, but it is impressive. Yeah, they do better than I thought they would.
DANIEL: What would human performance be, and then what would the model’s performance be, about?
JACK: Right, so human performance was around 95% at this matching task.
DANIEL: Okay, that’s good.
JACK: Yeah, it’s pretty good. I had to convince some of my coauthors to undertake the same task as the computer, so to undertake this matching task, because at this point, I’ve looked at the corpus so much that I basically memorized all of them. Humans get around 95% on this task, and there’s sort of two versions of this task that we consider. The first version is where we actually challenge models to do computer vision alongside the natural language processing. So, we don’t…
DANIEL: That was the pixel version, right?
JACK: Exactly. Yeah. So, the pixel version is you’re just given like the raw JPEG pixels of the cartoon and these five choices, and that’s what the model is given. So, it has to do object recognition and some sort of computer vision in addition to making all of these connections to the social norms and real-world entities. And in that setting, machines are getting around 62% or so. So, still a pretty big gap.
DANIEL: Even so, that’s not bad. Gosh.
JACK: Yeah, it’s shocking, actually. It’s funny. I started this project more than three years ago, so it’s been almost four years of working on this corpus. And in that time, a lot of stuff has happened in AI. And so, it’s been interesting to watch the performance of models slowly tick up and up over the course of me working on this project. So, yeah.
DANIEL: Yeah, gosh. Okay, there was the pixel version, but then was there also a text-based version? What was that like?
JACK: Yeah, so we have a second version where instead of the raw pixels, we substitute human-authored descriptions of the cartoons. And in that circumstance, we’re able to use models that don’t have image processing capabilities, so we can test giant, large language models like GPT-4, for example. And GPT-4 gets around 85% accurate, given a human-authored description.
DANIEL: And the human-authored description would be like, “Some men are sitting around with gigantic mugs of tea and coffee.”
JACK: Exactly. Yeah. Part of what I’m excited about with this work is we collect and release a big, annotated corpus of New Yorker cartoons. And we, in the pixel setting, use these annotations like the ones you’re describing to train computer vision models. But in the description setting, we circumvent that process by just handing the models the human-authored descriptions, and that corpus is available for download now. So, quick plug for that if you’re interested in this sort of thing.
DANIEL: Cool. We will have a link to that on our website, becauselanguage.com. All right. So, in this task where they had to look at a picture and say, “All right, given these choices, what’s the most likely winning caption?” it did okay. So, what was another task that you ran?
JACK: So, a different task that we looked at was a quality ranking task. So, the sort of matching-the-correct-caption task is kind of objective. Like in the ground truth caption contest corpus, there really was a caption written about this cartoon, and these other distractors really weren’t written about this cartoon. So, in that sense, it’s objectively attempting to reconstruct the data. The second task we considered is a ranking task, which is a bit more subjective. So, instead of this mismatch stuff, we give the model two captions, both of which were written about a given cartoon, but one of them was selected as really, really good, whereas the other one was sort of middling.
DANIEL: And it had to tell the difference between them.
JACK: Exactly. Exactly. Yeah. So, this is a more subjective task. I don’t like being prescriptive in telling people what is funny and what is not funny. But this was selected by either The New Yorker editors or by crowd voters as a really special caption for this particular cartoon. That was the second task we challenged models with.
DANIEL: 50-50 chance, but still a tougher task. So, how did it do?
JACK: To my surprise, not that bad. GPT-4, for example, gets around 65% accurate at this 50-50 task. So, not all the way to 100%, but in this case, we might expect the human performance also to be lower exactly because humor is more subjective.
DANIEL: That’s a good point.
JACK: Yeah. We have estimates of human performance, and it’s interesting. I think there’s a lot of variance in how well humans can do, particularly in reconstructing The New Yorker editor picks. I don’t know if you… have you ever tried entering The New Yorker caption contest?
DANIEL: I haven’t, but I’ve read a lot of them.
JACK: Yeah. It’s very, very challenging to win. I hadn’t entered myself prior to this project, and I’ve tried a few times since then. I’ve yet to win, so I’m still on the hunt. But I think one of our results that I found interesting was that at least for the annotators we considered, it was more difficult to reconstruct New Yorker editor preferences compared to crowd voting preferences. So, there is something…
DANIEL: Oh, that is interesting.
JACK: Yeah, there is something about The New Yorker editors perhaps that is difficult not only for my coauthors, the annotators and the human estimate to reconstruct, but also for people entering. I think many people have entered the contest since its inception a couple of decades ago and have yet to win. And so, I think New York editors have a particular style that they look for, and even so, their selections are often difficult to reconstruct.
DANIEL: Well, when a joke hits, there’s a rightness to it. It’s like, “Ah, yeah, it had to be that way. I wish I thought of that. “
JACK: Yeah. One contest that I entered, my first one that I entered, actually, after reading through so many of these in the process of doing this work, I think I was a little bit too heavy-handed. I made two puns, and I thought about it too hard somehow, and the result was a little bit heavy-handed. And one of the finalists was one of the jokes that I made, but it was much more subtle. And I think this gets into the realm, which I’m super happy to talk about, of what humor even is and what these experiments can tell us about it.
DANIEL: That’s kind of where I wanted to go. Like, what insights were raised by these experiments? Let’s delve into it. What is humour? When somebody walks down the street, it’s not funny. But when somebody slips on a banana peel, that’s funny. So, you could be tempted into thinking, well, the unexpected is funny, but it’s not just that.
JACK: Yeah, there’s a lot of nuance but I think the “unexpected” is a core piece. There are lots of theories of humor. I personally feel no single one tells the story of all humor. But there’s, for example, benign violation which posits that humor tends to arise from situations where there’s some violation of the norm — in the banana case, someone slipping — but it has to benign. So, this imagined person we’re thinking about, we know isn’t literally real. And so, the fact that this person slipping isn’t actually being harmed makes this violation benign. So, that’s one theory of humor.
There’s sort of a darker theory about humor. It’s sometimes called hostility or superiority theory. And this posits that sometimes humor comes from the darkest places within the human spirits. And ultimately, a lot of jokes are about laughing at someone with less than you and feeling better than them by laughing at them. I would like to think that this is not an all-encompassing theory of humor.
DANIEL: Well, but you can often analyse humour productively by thinking about who the target is.
JACK: Very true.
DANIEL: I just about got in trouble once in a class because you know those signs that say, “Local police are now targeting,” at least they have these signs around here, and it’ll say, “drink driving” or “speeding” or it shows what they’re looking out for so that you can not do that thing and not get in trouble with the police. Okay, so that’s the build-up. I showed this in class. It was, “Local police are now targeting… ethnics.”
JACK: Oh, my gosh.
DANIEL: Okay, so the reason I got in trouble in that class was that a few students sort of… they said that’s simply not funny! Targeting people of colour, targeting people of a different national origin. And I said, “Okay, okay, yes, you’re right. That’s not funny. But let’s look at the target of the joke. Can somebody please tell me the target of the joke?” Because these students of colour thought the target was them. But other students pointed out what I think is the intended reading. The intended target was police, because we make fun of what we suspect or what we know is a tendency… and this goes to very dark places because we’re taking something horrible and real and making them unafraid to put it on a sign. Like, “we know that we’re doing it and we admit that we’re doing it.”” [MAKES SQUIRMING NOISES] Targets.
JACK: I see exactly what you mean. I think dark humor in particular can be somewhat controversial because it is easy for people to have different perspectives about who the target of a joke is. I’ve run into this in my own life. I am personally a fan of dark humor and I often use it rhetorically in a self-deprecating way. But sometimes, you can get into trouble when you are intending to be self-deprecating but by bringing yourself down, you end up bringing someone else down with you.
DANIEL: Splash damage.
JACK: Exactly. And it’s something that I try to be cognizant of while simultaneously balancing, I guess, my natural state, which is, I think, to appreciate self-deprecation and dark humor, especially when my intent is to target only myself. So, it’s interesting. I think humor and many other much darker emotions are not so distant. People laugh a lot of the time to get over sad and stressful situations. It can be definitely a release of tension and I think this isn’t necessarily a universal trait. Not everyone laughs through the pain of different circumstances in life. But some people do. Yeah.
DANIEL: And then, you’ve got people who hide behind the joke. Like, they’re pretending that it’s a joke but they’re waiting to see what the reaction is and then when they get a bad reaction, they say, “Oh, it’s a joke.” So, you know, it’s the edgelord to fash pipeline which I think is really well established.
JACK: Yes. It is a very well-established pipeline. And I think that balancing being self-deprecating and appreciating the darkness in life and not trying to use dark humor to put people down but to uplift them is an art that I think ultimately very subjective. I think it’s definitely possible. But these tensions, I think, certainly exist. Yeah.
DANIEL: Yeah. Okay, so in the second task, the computer did okay at picking between the funny version and the not so funny version. Humans didn’t do so well either. So, bit of a matchup, a bit?
JACK: So, humans didn’t do so well at reconstructing The New Yorker editor’s judgments, but they actually did — at least the human sample we collected — fairly well at reconstructing the crowd voting judgement.
DANIEL: Yep, that makes sense.
JACK: Yeah. In conjunction with The New Yorker editor selections, The New Yorker runs a crowd voting process where people can vote on different captions. And our group of annotators, which were some of my coauthors in this particular experiment, were much, much better at reconstructing those judgments. So, they agreed with the crowd in, I think, around 84% of cases relative to this 50-50 baseline and GPT-4 gets around 73%. So…
DANIEL: Ah, that ain’t bad. That ain’t bad.
JACK: It’s not bad. Yeah. I’m impressed by that model. It’s pretty decent. We did have one additional experiment setting, which in some sense, I think is the most interesting one.
DANIEL: Mm?
JACK: We considered a case where we just challenged models to output a two- to four-sentence joke explanation. So, given the image and the caption, explain the joke.
DANIEL: Oh, okay. Now, I’ve got to say, I have had good experiences with ChatGPT in explaining why something is funny. Like, I’ve given it a few humourous situations, and it just hones in. So, what did you find?
JACK: Yeah, there’s this famous… maybe you’ve heard this E. B. White quote about dissecting humor.
DANIEL: Yeah, I have. [LAUGHS]
JACK: Yeah, we have this quote in the paper. Actually, it’s funny — I did some sourcing of this quote to try to find its very original form as part of the publication process of this paper, but for people who haven’t heard it, maybe I can read. E. B. White said in 1941, “Humor can be dissected as a frog can, but the thing dies in the process, and the innards are discouraging to any but the pure scientific mind.”
DANIEL: Yeah.
JACK: I think ChatGPT’s explanation here, while accurate, somehow deflates the humor. Never explain jokes, I think, is a mantra that is common among humorists and stand-up comedians and such. In this joke explanation task, we needed to curate a corpus of joke explanations for comparison, like human-written ones. But there’s no real, to my knowledge, experts in writing joke explanations.
DANIEL: No.
JACK: So, I decided, after some experimentation, to basically author a large corpus of joke explanations myself.
DANIEL: [CHUCKLES] Oh ho ho. Okay. Wow.
JACK: Yeah. I volunteered to write by hand over 600 of these things, and…
DANIEL: Wow!
JACK: I don’t know, so as a machine learning person, 600 doesn’t seem that many in terms of a training set size. So, I agreed to this in some meeting. I was like, “Oh, I’ll just write them.”
DANIEL: Augh!
JACK: What I didn’t realize is that 600 times two to four sentences turns out to be the length of a novella. And so, I have written, as part of this project, a novella that I would encourage no human to ever read because it’s very painful to go through and explain these jokes. And I have really, as part of this process, come to truly appreciate E. B. White’s sentiment that he expressed in this quote.
DANIEL: Oh, my goodness.
JACK: Probably the most painful annotation process I’ve ever conducted. But again, this is all available. I did it, so you don’t have to.
DANIEL: Oh, my gosh.
JACK: Yeah.
DANIEL: [FALTERINGLY] You are a machine! You have done a service to humanity. Oh, my gosh.
JACK: [CHUCKLES] I’ve done something, I’m not sure how big of a service, but hopefully others won’t have to do this. So, after curating this set of joke explanations, we did an experiment where we challenged exactly the setting you’re talking about. We challenged ChatGPT and GPT-4 to explain the jokes. And we did a blinded, AV human evaluation where we presented annotators with the machine-generated joke and the human-generated joke, and we asked them which was a more accurate, succinct, faithful explanation of the joke. And this is one of these things where you run the experiment and then you put it up for the raters and you close your eyes and you just hope that your joke explanations were good enough. And indeed, humans still win. My explanations were preferred in more than two-thirds of cases in a forced choice AV setting. So, humans are still succeeding at this joke explanation task.
So, yeah, in the paper we have some examples, but if you go through systematically the joke explanations from ChatGPT or GPT-4… I don’t want to say they’re in the uncanny valley because a lot of them really are quite good, but they do make subtle mistakes sometimes. So, a lot of the time The New Yorker captions are characters in the scene saying something. they have some trouble identifying who the speaker is in the scene.
DANIEL: Oh, yeah. And that would tip off a subject.
JACK: Exactly. Exactly. Sometimes, they simply miss parts of the scene that are important. So, GPT-4 in its current iteration, doesn’t have access to the raw pixels. So, sometimes they don’t understand that certain visual elements are actually more important than they are. There’s a really nice example where the cartoon itself — this one’s by Mick Stevens — it’s a couple sitting, having a glass of wine together, but in the upper right-hand corner of the cartoon, there is a pen that has broken the fourth wall, as if it’s the cartoonist’s pen. And it’s this very prominent pen. And the winning caption was, “My life’s a joke.” And the annotators, I believe, for this example, mentioned the pen as part of the cartoon annotation. But I don’t think ChatGPT necessarily keys into the fact that the pen is the most important piece. So, there is some modality bridging issue that I think is going to be tricky to solve, along with some of the other humor quote-unquote “understanding” gaps.
DANIEL: Okay, so humans still have the edge on humour, although… they’re catching up?
JACK: Yeah, it’s difficult to say. I think these are necessary, but not sufficient tasks. This goes along with my very neutral quotation of understanding. Like, certainly any entity that could truly capital-U Understand humor would do, at least for New Yorker cartoons, would do well at these tasks. But just because you do well at them, doesn’t necessarily mean that you capital-U Understand humor. So at least, for now, we’re still in a situation where the machine performance isn’t even sufficient. So, I have succeeded once again in circumventing the bigger sort of philosophical question.
DANIEL: Nice going. Let’s talk about the computer issues. When I was doing a lot of publication in natural language processing, it was all about n-grams and bag of words techniques. It was a really long time ago. But then, I started noticing things about embeddings and neural nets and deep learning and cosine similarity and stuff about… starting to recognise, “Oh, we’re defining words,” or, “We’re gathering statistics on words in relation to what words appear nearby,” and stuff like that. And now, large language models have hit in a big way. And I saw the bingo card from ACL, and one of the spots was, “Have you tried this using large language models?” [JACK LAUGHS] Is this the final stop on the road? Is this what we’re just going to use forever?
JACK: Yeah…
DANIEL: Or will something else take over later?
JACK: You know, it’s a great question. I think that for some lines of work, I’m not even sure this is a stop. There are some people still not using large language models. So, I want to give a shoutout to the folks who have picked a research agenda that have allowed them to circumvent this bingo card in a lot of ways.
DANIEL: Did they just back the wrong horse?
JACK: I don’t think so. I think that this philosophical question of: if a large language model can do well on a multiple-choice task, does it really understand things, I think this is a bigger question if your ultimate goal is to understand how humans use language. It’s not immediately clear to me exactly what you can learn about human use of language using just a large language model. And similar to the n-gram language models of the past — and maybe of the present too, maybe some people are still working on them — I think ultimately, it’s a lot of statistics, and we’re getting better at modeling these statistics. But this philosophical question about how much statistics can really tell us about language, I think, is difficult to circumvent even with the largest language models.
That being said, I’m cautiously optimistic about them in terms of their capacity to underlie useful language technologies. So by training, I’m a computer scientist, and so I’m interested in building tools that can ultimately help people interact with the world in better ways. And I don’t know — I think language technologies, there’s some unsung sort of applications of them that I think are quite helpful. So, indexing a database of documents for retrieving over, or Google Search — not that Google Search is unsung — but Google Search I think is quite helpful. Translation. I think all of these applications are pretty promising. And from that perspective, I think it’s difficult to not feel at least a little bit of excitement about these fraught, giant, problematic, but nonetheless somehow promising models. Yeah.
DANIEL: You say it’s fraught. What exactly makes it so… so fraught, do you think? What are the dangers here?
JACK: Yeah, so I think there’s a danger… Large language models aside, I think there’s a danger in applying automation in general. When you take a human out of the equation, I do think there is a fundamental difference in systems. So, there’s this great book, Automating Inequality, by Virginia Eubanks that isn’t talking about large language models but is talking about ways in which deploying much, much simpler forms of automation can nonetheless cause basically inequalities to exacerbate in the world. And I think some of these risks are just intrinsic to deploying automation. So, one has to be quite careful about when and where and how you’re deploying any sort of automation, be it some very simple tool, all the way up to a 175 billion parameter language model. So, there’s the automation deployment risk, I think.
DANIEL: Can we just talk about what exactly that risk is? I mean, the example that I’ll use with Dr Emily Bender is, “We reviewed your emails. It seems you have signs of depression as recognised by our model. You’re fired.”
JACK: Yeah. I mean, I wouldn’t want that world to exist. Yeah. I think that with automation comes many risks. I’m not saying, I mean… obviously, humans also can do bad things with power. This is maybe a large part of the story of the history of humanity. But yeah, I think automation has unique challenges. Like, there’s this psychological phenomenon. I think it’s called sometimes automation bias, where even well-intentioned humans can often ascribe too much confidence to a decision if it’s being made by some automated tool, I believe. Yeah, so automation bias, I think, is one particular risk of automation that, I think, is specific to it.
So, yeah, I think with respect to large language models, deploying them is tricky because ultimately, they’re probabilistic models over language and you can’t 100% guarantee what they’re outputting a lot of the time. Like, you can do mitigations and you can make statistical arguments like, “Oh, we did a million samples and only one of them was detected as dangerous.” But still, you know, that one could be quite important. So, deploying any statistical method, I think, is particularly tricky. So, I think weighing the pros and the cons for any given scenario is important.
One example that I… so, my research is mostly in multimodal language technologies, which is how I actually came to study The New Yorker caption contest.
DANIEL: Oh, so like, text and vision and gesture.
JACK: Yeah. So, most of my work is in vision and language. So, trying to combine something like ChatGPT or GPT-4 with a visual input. And one of the most, in my opinion, promising potential use cases for vision and language models is to improve web accessibility. The web has a lot of images, and these images are inaccessible either to low vision and blind users of the web, or to folks living in places where there’s not fast enough internet access to download the images. And so, how do you generate a description of these images such that you’re providing an equal experience to the folks who can access the images? And in some sense, this problem is very, very hard because images themselves have their own pragmatics. Images are often used for a communicative purpose online. And so, simply substituting in, you know, even an accurate description is oftentimes not quite enough because there’s so many ways to describe an image.
And yet, despite all of these hurdles and the risks of outputting the wrong alt text that actually is misleading, some work in human computer interaction suggests that as a first step, some of the automated tools we have that can do captioning are indeed potentially helpful for this task of alt text generation. So, I think pros and cons exist with deploying and developing this sort of technology. Maybe it’s because of my training, but I am cautiously optimistic about at least some of the use cases.
DANIEL: Can you tell me something that if I made you King of Technology [JACK LAUGHS], what would you tell people you’re not allowed to do?
JACK: So, I’m the king of technology and I can write laws and people are going to obey them. Wow. My first inclination is, I don’t want this power! But… um…
DANIEL: Well, you’re allowed to prevent people from doing a certain thing. You’re not allowed to… do what? When you’re making these systems, here’s what you can’t do, because we’re trying to build the world we want here.
JACK: Sure. I think a rule that strikes a reasonable balance between risk and potential benefit is you’re not allowed to not listen to people. So, you, I believe if you’re deploying one of these language technologies, should be open to critique and criticism. And I think that if I were the King of the NLP World or something, I would encourage folks or… I’m the king, I don’t have to encourage. I would tell folks they have to take feedback seriously. Even if they aren’t affecting negatively people who are directly using their system, I think that listening and iterating is a bare minimum baseline for deploying language technologies. So, maybe I’m not a very forceful king or something, but that would be my first rule: Listen.
DANIEL: No, I like that. It’s table stakes, basically.
JACK: Yes, table stakes. Exactly. Yeah.
DANIEL: You must be running across a lot of people with ideas about AI and some naïve opinions. What are people getting wrong about it? Are there any sort of things, ideas that you have to push back on over and over again? What are you hearing?
JACK: Yeah, I’ll give a few examples. So, I think a lot of these large language models, people talk about behaviors that are emergent. Right? So, these are behaviors that maybe you wouldn’t have expected GPT-3 or -4 to be able to do a priori. And yet, it can kind of explain jokes and for any given task it can do kind of okay at this. I think one potential misconception that some have is I think people underestimate just how interesting and weird the internet is. Like, I’ve seen many examples where someone describes some purportedly emergent behavior to google it and find out actually there is a whole community of folks on some blog from 2007 who have been explaining jokes, or a whole community. Like Reddit, for all of its folly in terms of pretraining data, I think there are a lot of really cool niche subreddits that support a lot of different types of communication.
DANIEL: That’s true.
JACK: And I think people underestimate the breadth of the web. People talk in terms of how many billions of tokens have these large language models seen? I think they’re getting into the trillions of tokens and this number is almost unfathomable! Like, I don’t have an intuitive connection to the number one trillion. And I think there is some disconnect between what behaviors people call emergent and what actually maybe already exists on the web.
I should caveat this by saying, I do think these models are generalizing in a new way. So, I don’t think they’re purely memorizing. I should highlight, one very surprising thing to me that kind of is yet unexplained about these large language models — maybe I’m just looking in the wrong places — but these models are trained to predict the next word, right? in trillions of web tokens, at least at the start. First, it’s super unclear to me why predicting the next word would give rise to linguistic representations that can actually do a very broad range of tasks. Like, a priori, I wouldn’t have guessed that predicting the next word was such an expressive task that actually yes, this is encapsulating a lot of… at least operationalizing a lot of what we want to do with language technologies. It’s very surprising to me.
DANIEL: Yeah, that is weird.
JACK: It’s very weird.
DANIEL: You know what’s weird to me? It’s able to put out stuff that’s very surprising. It’s able to put out grammatical output. It’s able to put out sometimes useful output. And the ability to do that was in the text the whole time. We talk about, “Oh, no, you need real-world knowledge.” “Oh, you need to have a body.” “Oh, you need to have social relations.” Nope, all that stuff was in the text the whole time.
JACK: It is really quite amazing that you can get so much from just predict the next word. And I mean, it’s not just predict the next word in terms of GPT-4. So, GPT-4 has had some additional training on top of it with some reinforcement learning. I won’t get into the details there. But yeah, it is just very surprising. One other thing that maybe I’ll highlight is… so, I think large language models again have a lot of risks and downsides, but also from… So, I studied machine learning in grad school, and so I’m a computer scientist of that flavor by training. And there is something, in my opinion, quite interesting going on with scaling at a theoretical level. So, I think… it’s very shocking to me, actually that… so, in machine learning class, you learn about this concept called overfitting, which is…
DANIEL: Yes. Yes.
JACK: …if you make your models more expressive, they might memorize your data instead of learning generalizable patterns from it. So, that’s a core principle in classical machine learning.
DANIEL: And it’s bad if you’re just simply memorising all the training examples, because then the output is just going to be what you fed into it. You want it to be able to do more than you’ve fed in.
JACK: Exactly. That’s exactly right. Generalization is largely the goal of machine learning. So, carefully balancing learning patterns from the data, but not memorizing the data, is arguably the fundamental challenge of machine learning. And in school, I was taught, “Don’t make your models too expressive, don’t make them too many parameters because they’ll just memorize.” And yet, we’re seeing this weird counterphenomenon with scaling, where actually, by adding more and more parameters, you’re overfitting less, you’re generalizing better. These more expressive models are acting opposite of what I was taught in machine learning class. And so, I do think that while it is correct to say that, “Oh, you know, OpenAI has all these GPUs and this is problematic in a lot of ways,” I do think there is something fundamentally interesting going on with respect to generalization that has to do with the number of parameters and the number of flops these models have that I have yet to see a great explanation of. And so, I do think there is something kind of interesting going on there. Yeah.
DANIEL: Mm. The working title for this episode is “AI Hype Hosedown.”
JACK: Okay. [LAUGHS]
DANIEL: What AI hype would you hose down?
JACK: Um… gosh. I would bring it back to the corpora these things are trained on. I think in addition to folks underestimating the breadth of what exists on one trillion internet tokens, I think a lot of these systems, like ChatGPT and GPT-4, for example, are trained on additional human supervised data. I think in a lot of discourse, there’s this conflation of emergent behaviors with all this mystery about generalization versus GPT-4 was trained with a lot of additional hidden human supervision. So like, okay, you can ask it to generate a poem, and you might not have expected an annotator to ever have tried to write a poem out by hand such that GPT-4 could generate it. But I wouldn’t be surprised if in this hidden supervised dataset, there is actually some poem generations that annotators have been paid to author.
DANIEL: Interesting.
JACK: I have no insider info, so I’m not saying specifically this example, but there’s a lot of human supervision that goes into these machines. Yeah.
DANIEL: Okay. I guess I got one last question. We started out with the discussion of your title, Humour “Understanding”.
JACK: Yep. [LAUGHS]
DANIEL: And all through this discussion we’ve been putting “understanding” in air quotes. But I’m also aware that natural language understanding is a real goal that a lot of people are working on. And I’m with you. I don’t think that large language models “understand” language in the way that a human does. That being the case, what’s the path to natural language understanding? What more would we have to add to a large language model to get understanding? Or is that even possible? Is that a fool’s errand?
JACK: I think it comes down to what one means by understanding. I certainly think that models will get better and better at doing different evaluations we throw at them. But if a priori one has determined that for their own definition of understanding — like machines simply can’t — that’s fine and defensible. I think to go beyond our current large language models, I think there’s a lot of different things we can do. I think some folks talk about world models where, in addition to some giant neural network, you also have an explicit representation of the world and the state of the world, and you have the capacity to interact with that world and do “experimentation” quote-unquote with your model in a world. So, some people are working on this. Particularly in the reinforcement learning community, this is popular.
I am someone who is a vision and language researcher, so I believe that expanding out these models with broader views of reality will ultimately lead to more promising language technologies. So, adding in images, adding in audio, adding in video, I think these all can help expand out models’ capacity to “understand” the world. I’ll let you guess whether or not I put air quotes on that “understand””. [DANIEL LAUGHS]
And I don’t know. There’s lots of unsolved practical problems as well. So, the risks of deploying automation, like we talked about, are risks… I’m not a lawyer, but there’s a lot of legal questions about whether or not it’s appropriate legally to train on people’s data without their explicit consent. I think this is something, is this fair use? Is this not? I’m not a lawyer. I’m not sure. I think this is another interesting avenue that needs to be hashed out. So, yeah, I’ve once again circumvented your question about “understanding” though. [LAUGHS]
DANIEL: [CHUCKLES]
JACK: I think it comes down to a philosophical question. I think if you believe that only humans can understand things, I think that’s a completely defensible position. But I don’t know. I’m a computer scientist, I want to build helpful tools. This is above my pay grade. I’m not smart enough for philosophy.
DANIEL: We’re talking to Dr Jack Hessel of the Allen Institute for AI. Jack, how can people find out what you’re doing?
JACK: Yeah, so, I have a website. It’s jmhessel.com. I’m also on Twitter, probably too much, the same handle @jmhessel at Twitter. And yeah, thank you so much for having me on, Daniel. This was a lot of fun.
DANIEL: Well, thank you for sharing your advice and your insight. This has been really fascinating, and I hope a lot of people out there will find it edifying as well.
JACK: Oh, thanks again. Yeah.
DANIEL: And now, it’s time to play our favourite game, Related or Not.
BEN: Yesssssss…
DANIEL: When I was in the USA, on holiday in my hometown of Spokane, Washington — actually, Cheney, Washington, but everyone from Cheney says that they’re from Spokane because nobody knows where Cheney is — I got the chance to take my two darling daughters to the famous and historic Looff Carousel.
BEN: Loof… spell.
DANIEL: L-O-O-F-F, Looff.
HEDVIG: That sounds like it’s a name.
DANIEL: It is. All 57 of the animals were hand carved by hand carver, Charles Looff, around 1909. He made 40 carousels in his lifetime, and there are 10 existing, and one of them is in Spokane.
HEDVIG: Can I guess what the words are going to be for Related or Not given the information I have so far?
BEN: [LAUGHS]
DANIEL: Go on then.
BEN: This is a fun game.
HEDVIG: Is it going to be CAROUSEL and CASSEROLE?
DANIEL: You’ve got one of those right.
HEDVIG: Okay.
BEN: Is it CAROUSEL and CAROUSE?
DANIEL: Actually, Ben is closer.
BEN: Okay.
DANIEL: So, let’s see. I had a question about the word CAROUSEL. I’m actually going to give you not two words, I’m going to give you three words, one of which is the most likely to be the right one. And you have to guess which of my three answers is most closely related to CAROUSEL.
BEN: Oh, okay. It’s a multiple choice. Cool, cool, cool.
DANIEL: This one’s multiple choice. But before I give you the three answers, Ben, you’ve already guessed CAROUSE, and that is one of the choices. What do you think the relation is there?
BEN: Um, well, carousing is kind of carrying on a bit. Right? Like, if someone is carousing through the town, they’re having a good time. And, you know, carousel is a thing on which you sit and go up and down and have a good time. Those two things would be good. Or does carouse have, like, an explicitly sexual dimension? Have I misunderstood that?
DANIEL: I am unaware of any way in which CAROUSE has been used sexually, but what hasn’t, right?
HEDVIG: But you are also of Mormon extraction, so…
BEN: Drink alcohol and enjoy oneself and others in a noisy, lively way. Okay, cool.
DANIEL: Okay. Hedvig, now I’m curious as to what relation exists in your mind between carousels and casseroles. Was it just word resemblance… ? Or have…
HEDVIG: No, no. It’s to do with…
DANIEL: Is there a story?
HEDVIG: No. I thought it was… they’re all related to this French word that means like a round thing going around, and then you boil, because in a lot of other European languages, KASTRUL is the word for pot. Like, when you boil water.
DANIEL: It is kind of a pot, isn’t it?
HEDVIG: Yeah. The animal you call sperm whales, we call KASKELOT. And I think it’s also related because in their head, they have a bunch of oil, and the oil separates in the same way that oil does when you boil it. So, they’re pot whales. Anyway! Long story. Okay, can I guess at the other words? So, you have CAROUSE. The first word, we’re going to guess… what’s another /k/ word that Daniel could have put in there? Not CASSEROLE or CAROUSE. CAVORT? No, that’s not a word.
DANIEL: Oh. Okay. It’s got a /k/ in it. Well, I’ll give you the three choices.
BEN: Okay, come on, yeah, lay it on us.
HEDVIG: Okay. never mind. I suck at this game. Give us.
DANIEL: Okay, well, keep in mind that a CAROUSEL is a bit like a chariot. It’s got animals, pulls you along, it moves. And CHARIOT has a relation to Latin CARRUS. So, is it from CAR, which in turn comes from chariot? Is it related to CAROUSE, which you do when you’re having a good time? Or, is it modeled after jousting? You see, when people are feeling quarrelsome — in Italian, GAROSELLO, a little quarrelsome — you might joust with them on horses. And the carousel is modeled after this kind of fight when people are feeling quarrelsome.
BEN: I like that one!
HEDVIG: I don’t like that one.
DANIEL: So, the three choices are: is CAROUSEL related to CAR? Is it related to CAROUSE, or is it related to GAROSELLO, which means a bit quarrelsome.
BEN: I’m coming in first.
HEDVIG: Okay, go.
BEN: I am going hard for option C.
DANIEL: Okay.
BEN: GAROSELLO. And the reason I’m guessing that is not because the two words are the most similar sounding, though also that, but because carousels are almost universally done up in that really twee, gaudy, like Renaissance shit, that is like just all the rage throughout the Mediterranean. So, I’m thinking, just purely looking at the little ponies on carousels, I’m like: that is exactly the kind of shit you see in, like, chateaus and fucking…
DANIEL: It’s that whole Pre-Raphaelite thing, wasn’t it?
BEN: Yeah. Like, with gilt on everything and just all that kind of stuff.
DANIEL: Okay, we have a vote for GAROSELLO. Hedvig, what do you think?
HEDVIG: I think the order of these items betray Daniel’s research process.
[LAUGHTER]
HEDVIG: And I think that the third one is reaching. I think that word in Italian is related to war, like GUERRA something, and I don’t think it’s related to CAROUSEL, I think it’s number one.
DANIEL: The car.
HEDVIG: Yeah.
DANIEL: With the side glance at chariot.
HEDVIG: I think it’s boring. Yeah, I think it is that.
DANIEL: Ah, we do know that if a story is really interesting, it’s probably wrong. The correct answer: the idea that it comes from GAROSO or GAROSELLO, a little quarrelsome, the OED says that this is “doubtful””, and the right answer is probably the boring one. It’s a car, it’s a carosello. It’s a little chariot.
BEN: Hang on. Hang on. I’m not liking this, probably. I’m not liking this unlikely and probably. I want: this is what it is.
DANIEL: I want hard science here. This is etymology, damn it. We know these things.
BEN: Okay. Damn.
DANIEL: So, Hedvig, you got this one. Congratulations. Take a victory lap. On a carousel.
HEDVIG: Pew, pew, pew.
DANIEL: I was surprised to find that CAROUSEL is entirely unrelated to CAROUSE. It comes from French carouser, to drink or to quaff, and it gets it from German gar aus, which is quite out, to drink out. You’re drinking out, man.
BEN: That’s fun.
DANIEL: Yeah, that’s carousing.
BEN: Okay.
DANIEL: A lot of people have been sending us these puzzles. We’re really enjoying them. Thank you for sending them. Keep sending them through the usual channels, and we will feature them on a future episode.
[INTERVIEW BEGINS]
DANIEL: I’m here with Dr Emily Bender of the University of Washington Linguistics Department. Hi, Emily. Great to talk to you.
EMILY: Hi. I’m excited to be on the show for the second time.
DANIEL: Second time, because the first time it was in Melbourne at the ACL 2018. Uh… a lot’s happened since then.
EMILY: A lot has happened since then. A whole pandemic. [LAUGHS]
DANIEL: I was thinking of that, but I was also… because at the time, we were talking about your book, Linguistic Fundamentals for Natural Language Processing: 100 Essentials from Morphology and Syntax.
EMILY: Yeah. And possibly the semantics and pragmatics one, which was in process. So I think you had come to my tutorial based on the second book.
DANIEL: That’s right.
EMILY: We were talking about that, yes.
DANIEL: Oh, we were talking about that and that hadn’t come out yet. Everything about that blows my mind. But now, we’re in kind of a different zone now, and you’re doing a lot of different stuff.
EMILY: Yeah.
DANIEL: Large language models have landed. It’s been about a year since we’ve had the various flavors of GPT going around. Everyone’s freaking out. It’s like, “Oh, my god, this is amazing.” “Oh, my god, this is terrible.” “Oh, I’m so scared.” “This is going to kill my job.” “This is going to make my job way easier.” I’ve seen that you’ve been doing a lot of raising the flags and hosing down a lot of hype. Would you say you’re doing more hosing or more warning?
EMILY: I think in minutes spent doing it, more hosing. In the sort of where I want to put my intellectual effort, more warning. A little bit off on the timeline, actually. So, GPT-2 was around in 2019.
DANIEL: It’s an old one, isn’t it?
EMILY: Yeah. And that’s when Alexander Koller and I wrote the Octopus Paper, which was published in 2020, and shortly after we published that, GPT-3 was released, and people were playing with it. But it didn’t become really a big thing outside of tech circles until ChatGPT landed, and that was November of ’22.
DANIEL: Because people could play with it then.
EMILY: Yeah.
DANIEL: And that’s when it really… It was like a bomb. It just exploded onto the scene.
EMILY: Yeah, absolutely. And it turned into… it went from this conversation that was sort of in tech circles to everybody wanting to talk about it.
DANIEL: We’re going to talk about the octopus.
EMILY: All right.
DANIEL: I want to talk about the octopus, but let me just start off by asking, where are we now? [EMILY LAUGHS] Who are the players that you’ve noticed and what are the latest developments here?
EMILY: Yeah, where we are is in a place where there’s a lot of effort and power concentrated with big tech companies. So, you know, OpenAI is the one behind the GPTs and they are absolutely in bed with Microsoft. Microsoft has invested billions in them and incorporated something like ChatGPT in their search interface.
DANIEL: That’s been going well.
[LAUGHTER]
EMILY: So, speaking of papers we could talk about, in 2022 with Chirag Shah here at the Information School, I published a paper on “Why you would not want to use a chatbot for search.”
DANIEL: What?
EMILY: Yeah.
DANIEL: That sounds… oh, man. That was, like, one of the use cases that I thought was the most solid.
EMILY: It is really, really a bad idea in many levels and we published that paper because Google started making noise about that in late 2021, in fact mid-2021. And so, we looked at that and said, “Well, that’s a terrible idea,” and wrote a paper.
DANIEL: Wow.
EMILY: And then, that’s happening. Like you were saying before, warning, yes, that’s where my paper writing is going, but sort of like day to day talking to the media on social media, it’s about hosing. So, where are we? So, Google’s big into this. The Microsoft-OpenAI partnership has sort of forced their hand. You also have a bunch of smaller players who I think some of them are really into, “Let’s find the useful aspects of this technology and make it widely available.” So, I think that’s what Hugging Face is trying to do. Unfortunately, there are also bad actors who want to take advantage of things like Hugging Face to create a platform for some really awful stuff.
DANIEL: Let’s talk about the bad actors.
EMILY: Yeah. So there’s this basically machine learning shock jock named Yannic Kilcher, who is like a YouTube personality, and he trained a version of GPT-3-ish sort of finetuned on 4chan.
DANIEL: Gross.
EMILY: And yeah, published that through Hugging Face as GPT-4chan, which did what you would imagine it would do, and that since got taken down. But there’s…
DANIEL: Really?
EMILY: Yeah.
DANIEL: That actually surprises me.
EMILY: So, there’s some great folks at Hugging Face. I’m thinking of, for example, Meg Mitchell, who are really thinking hard about, how do we do this in a beneficial way while actually mitigating risks? And that’s Meg Mitchell’s whole career, is sort of pro-technology, like, “Let’s make this stuff work, but let’s make it work well by thinking carefully about risks.” And so, Hugging Face got it together basically and took that down and has been developing policies so that they can be more proactive rather than reactive in the future. So, that’s like sort of a brief overview of the landscape on the language model side.
Of course, there’s all this stuff about image generation, which is part of the same conversation. I’ve taken to talking about synthetic media and synthetic media machines, or text or image synthesis machines. I like to talk about the output of these things as being “extruded” from the text synthesis machine.
DANIEL: That’s a good way of putting it. Squirted out through a tiny hole in the pipe.
EMILY: Exactly. And EXTRUDED is kind of a gross word, isn’t it?
DANIEL: I like it!
EMILY: Yeah. But something that’s been extruded isn’t really appealing.
DANIEL: Come onnn, it’s tasty. Whenever you watch these How They Make It shows, there’s always an extruder.
[LAUGHTER]
DANIEL: There’s hot dogs, chicken nuggets, vegetarian chicken nuggets wouldn’t exist if not for extrusion.
EMILY: Right. And that’s not the most appealing part of our food system.
DANIEL: Oh… you’re right. It’s tasty but it’s not good for us?
EMILY: Tasty, but not good for us. And you don’t want to see how the sausage gets made.
DANIEL: Oh-kay. All right.
EMILY: [LAUGHS]
DANIEL: I feel like at this point, since we are speaking together, I feel like just mentioning that in this discussion, we are largely talking about English.
EMILY: Yes, thank you. We are largely talking about English. The very large language models actually do have multilingual training data inside of them. I think they are most widely used for English. I think they have been most widely tested, to the extent that they’ve been tested at all, in English. And so, usually when people talk about applications, they’re talking about English. And there’s some real downsides to actually the multilingual side of it. So, there is somebody who is affiliated with the Distributed AI Research Institute. And I’m feeling bad that I can’t think of his name off the top of my head, but he has, in addition to that affiliation, works on machine translation for languages of Ethiopia in, I think, a small company and has found it hard to get funding because Facebook has told the world that they have solved low-resource machine translation.
DANIEL: Yeah, that’s not true.
EMILY: Yeah. Doesn’t work well. As a speaker of those languages, you can say this does not work well. But the VCs hear Facebook, so why are we investing in this?
DANIEL: I love the idea. Is their heart in the right place or are they trying to cut it off at the knees?
EMILY: I think they are Columbusing it.
DANIEL: Yep. Okay.
EMILY: They think they’re doing something good. It’s well intentioned, but it doesn’t actually involve the communities that they are ostensibly serving.
DANIEL: Ah, see, that’s an old problem, and we know about that one.
EMILY: Yeah.
DANIEL: They shouldn’t have gotten tripped up on that one.
EMILY: No, they shouldn’t have.
DANIEL: Are we just saying the same thing? We’re not stochastic parrots, but are we ideological parrots?
EMILY: We’re not parrots of any kind.
DANIEL: Okay.
EMILY: And so, let’s talk about stochastic parrots. So that phrase, first of all, no shade on parrots. Amazing birds.
DANIEL: Birds are great, smart birds.
EMILY: Parrots are great. The word PARROT and stochastic parrots really draws on the English verb to parrot, which is about repeating without understanding. And when we take pieces of ideology and sort of repeat them or rework them or come back to them, the key thing there is that it’s about the meaning as well as the form and not just the form. So people often say to me, “Don’t people just go around sort of just saying the same thing over and over again?” It’s like, “Well, yes, but 99.99% of the time, it’s actually the meaning that we’re drawing on.” Sometimes with the form, that’s the whole thing about memes. My one example for a time where you might say something where you don’t really have any meaning is if I say: Hey, Daniel, how are you?
DANIEL: I’m good, thanks. How are you doing?
EMILY: I’m fine, thanks.
DANIEL: This is pretty… I think I’ve said this before.
EMILY: Yeah. Yeah. Exactly. It’s one of those cases where you probably would say exactly the same thing no matter how you are. It’s just the rote greeting.
DANIEL: It’s phatic.
EMILY: Yeah, exactly. Exactly. And I love there’s a version of it in Japan. I think it’s a bit archaic now, where instead of, “How are you? I’m fine,” it’s, “Where are you going?” “Oh, just a little bit that way.”
DANIEL: That’s also in Australian languages too.
EMILY: All right. Cool.
DANIEL: Off in the medium distance. And you’re not going anywhere. You’re just sitting there.
EMILY: Yeah. [LAUGHS]
DANIEL: Just like I’m not fine. Okay. But that happens… I mean, a computer could duplicate that. But what’s missing is that I’m doing it in a situation where I intend certain things and I want to make certain things happen.
EMILY: Yeah. So, let’s roll back to that conversation that prompted you to say this when we were talking about how Facebook has hit the same problem again about Columbusing, and you said, “Are we just rehashing the same ideology?” And you were referencing the fact that we have come back to a conversation that you and I have not necessarily had together, but each of us have had with many other people many, many times. And there, what mattered was the content of the conversation. It wasn’t phatic.
DANIEL: Yeah. It wasn’t at all, because I have knowledge of what you do in your time, and we know certain things together. Certain things are on the common ground, even though we haven’t actually had this conversation before. So, I had an idea in my mind that it was likely that you would know about the kind of conversations, because I know you have them, and I know that about you. So, we’re pulling in a lot of stuff about common ground and guesses about each other’s knowledge, and all the stuff that we talk about that computers aren’t doing.
EMILY: Exactly. And communicative intent. So, you were saying that, not just to make some sound for the recording, but to communicate something in particular drawing on that common ground and to lead me to have certain beliefs about your beliefs, etc.
DANIEL: Yeah. And then, we can put those on the common ground, and then we build and we’re building a conversation. The other thing that I think language models aren’t doing at all… we had a chat with Dr Nick Enfield a while ago about his book, The Consequences of Language. One of the things he mentions is when we agree to sit down and have a conversation, we take responsibility for making the conversation go well. We have a responsibility to each other. I don’t fly all the way over here, and you say, “Come on over to my office, we’ll have a chat,” so that you can go, “Ugh, I don’t want to talk to you,” or, “I am not prepared,” or something like that. That’s not…
EMILY: Right.
DANIEL: We owe each other something just by the fact that we have decided to come together and have a conversation.
EMILY: Exactly.
DANIEL: Computers have no obligation in that way.
EMILY: No. They have no accountability. They have no public commitments. They have no relationality. It’s just extruded text that, because of the way current technology works, looks really, really plausible and really, really convincing.
DANIEL: Yeah, it is. It’s really convincing. I mean, when you throw in the anthropomorphic fallacy where we live in a world of speaking humans, and so if it generates text, we think, “Oh, well, it knows stuff.” Well…
EMILY: And the companies designing it are leaning way into that. So, there’s this great recent paper, pre-print, not yet peer reviewed by Abercrombie, et al. And they have this wonderful example where they say, “Okay, let’s take the output and show what it would look like if it were de-anthropomorphized.”
DANIEL: De-anthropomorphised.
EMILY: And I have to say, I have a little bit of ire for this whole situation, making me say the word “anthropomorphized” over and over again, worse spelling it. It is so hard to write that word!
[LAUGHTER]
DANIEL: You have to decide whether it’s I-Z-E or I-S-E.
EMILY: But also how many P’s and how many H’s and how many H’s… how many syllables? So, it’s something like the user gives the input and I’m trying not to say the user asks because I don’t want to describe this as a conversation.
DANIEL: Not a speech act.
EMILY: Yeah, well, the user’s speech act maybe is, but on the computer’s side, all it’s getting is input. And the input is something like, “Don’t you love the feel of sun on your skin?” And the computer’s output, which is not answer, it’s just an output, is something like, “As a large language model, I actually don’t have skin and therefore can’t understand what it would feel like,” etc. etc. “But from my training data, I know that…” etc.
DANIEL: That’s a good answer.
EMILY: It could be much, much better. So, that’s actually the original answer. And their de-anthropomorphized answer is, “This system is a large language model and does not have a body, therefore it has no feelings about sun or skin,” etc. “From its training data…” No, it’s even better than that. It’s like, “According to its training data, many people enjoy the feeling of sun on their skin.”
DANIEL: That’s very good.
EMILY: So, it’s removing the I. It’s removing “understand”. It’s removing this illusion that there is a perspective there that the computer could possibly be taking.
DANIEL: That is appropriate.
EMILY: Yeah. And we do not see very much of it.
DANIEL: One of the talks that you’ve done recently is “Resisting dehumanisation in the age of AI””. We know some things about what large language models are and aren’t doing. We know that they don’t understand language. They hallucinate answers. They give wrong information. They’re not using language like we do. They’re not learning language the way children do. All they’re doing is looking at the input and deciding the most likely output. But one thing in your talk that surprised me was the idea that to say that these algorithms are doing language like we do is to deny something about the humanness of what we’re doing, which is things that we’ve been talking about, like interacting together, having intersubjectivity, and all of that. So, dehumanisation is a problem?
EMILY: I really think it is, yeah. And that’s one kind of dehumanization that’s coming from the people selling AI. And it’s a little bit annoying and irritating, just as a human who is frequently the target of the question, “How do I know that you’re not a stochastic parrot?”
DANIEL: Yep.
EMILY: But it can be much, much worse than that. If you look at the way that so-called AI technology is being used to displace workers and deskill jobs, it’s basically saying, “Look, humans just do computation like this, and so let’s have the computer do a big chunk of it, and then we can do cleanup with the humans and pay them less because that’s cheaper.”
DANIEL: Okay.
EMILY: So, if we look at humans as computing machines, that is very dehumanizing. And the more we look at the large language models and say, “Well, that’s just what people do,” the more we’re feeding into that other narrative as well.
DANIEL: Yeah, okay. We’re feeding into a lot of other narratives as well. Like narratives… we know that large language models are biased because they’re pulling information from our biased data because we’re biased. So, we’re seeing things like male bias or white bias or upper-class bias, because the white mainstream English that it’s trained on is coming out. Here’s a question that I get a lot. If everybody is using large language models like the various flavours of GPT, is it going to somehow iron language flat and reduce the amount of variation in the language that we then use with each other?
EMILY: So, I guess if we ended up in a scenario where everybody was using it as a writing aid, then yes, we would lose some of the variation that we see, some of the richness of language. I would be surprised if that flattened out spoken language very much. And in fact, it might go the other way. It might be that as people work to prove that they are human in their writing — and this is a question that I’ve been asked is, “Okay, given that we have all these synthetic medium machines, how do we prove authenticity anymore?” And I don’t know how to do it in an ironclad way, but one thing is to really show that you value it and, as a news organization, say, basically put the reputation on the line saying, “Nothing here was written by a text synthesis machine,” and state that as a value.
But I also think that people might start adding rhetorical flourishes, turns of phrase, things that sound very much unlike ChatGPT in order to stand out and make it clear this came from a person.
DANIEL: I’ve argued that it wouldn’t change language in any particular way. Someone once asked me: “When I talk to my phone doing speech to text, I. Talk. Like. This. So. That. It. Will. Understand. Me. Do you think people will start talking like that?” And I say, “No, we talk like that for a purpose.” And then, when I got a different purpose, I talk a different way. It’s not going to somehow make me forget to talk normally.
EMILY: So, this is something I would love for somebody to look into, and I haven’t. But have you noticed the way YouTubers talk?
DANIEL: Yes, there is a YouTube accent.
EMILY: Yeah.
DANIEL: But that’s social too.
EMILY: Right. But I’m wondering if that is not a little bit technology driven. To me, it sounds like something that might remain comprehensible when sped up or something.
DANIEL: Wow. We need to do the work.
EMILY: Yes, someone needs to pick up this research topic. Like, where does that come from? And what are the technological parameters that might be pushing it in one direction or another? But certainly, also it could be that some YouTuber started doing something for a technological reason and then that became the way you talk on YouTube and then it spread sort of socially would seem really plausible.
DANIEL: It would, wouldn’t it? It totally would because we’re just these unconscious social pattern absorbers.
EMILY: And then, another kind of speaker that you have to keep in view is, what about people who have to code switch in order to get technology to work for them?
DANIEL: Yeah, Australians.
EMILY: Australians. People who use African American language, for example. My in-laws, who are from India, for a long, long time couldn’t get the in-car navigation systems to work for them. So, there’s a huge amount of accent discrimination that’s going on. If you are using the technology regularly and you have to code switch into what’s not your sort of most authentically self accent, those language behaviors might then feed into language change in interesting and unpredictable ways.
DANIEL: Possibly. I sent over some questions earlier and one of your answers surprised me. Here’s what I wrote. “This technology can be quite useful if people are appropriately skeptical and cautious.” And I thought that seemed uncontroversial.
EMILY: [LAUGHS]
DANIEL: You disagreed.
EMILY: Yeah, I disagree. And this is something every time I talk to the media, people want to get me to say, “What are the good use cases of this?” Or, they’ll start with these, like, “This can be beneficial.” And I’m like, “Why do we have to presuppose that? Why is that just given?”
DANIEL: But it… but it… but it is useful!
EMILY: [LAUGHS] What’s it useful for?
DANIEL: Okay, so I did a thing where I was going on the radio, and I had this linguistic term that I needed, and I said, “Ugh, what’s the name for that linguistic pattern where nouns get stressed on the first syllable and verbs get stressed on the second one, like OBject and obJECT?” And it said, “The linguistic pattern you’re referring to is called initial stress derivation in this pattern,” blah, blah, blah, and it explained it. So I went, checked it. All right? Initial stress derivation. Yep, that’s what it is. That was pretty useful. I can go to it, and I can say, “What’s that movie from like 1983 where I think it was Lenny von Dohlen is a computer and he falls in love with a girl, and what’s the movie?” And it’s like, “Oh, that’ll be Electric Dreams.”
EMILY: And then you can go back and check it. So, this sounds like you’re using it for a certain kind of information access. That’s what we use search engines for, where what’s missing is the name. What’s missing is what would be the search term. And then once you have it, you can use that as a search term and go back and verify.
DANIEL: Right.
EMILY: All right. That sounds like a plausible one. So, I’ve done some thinking about this. Like, what are the beneficial use cases of synthetic text extruders?
DANIEL: Yep.
EMILY: Right? And I have some criteria for what makes a good use case.
DANIEL: Oh, please.
EMILY: So, it has to be a situation where what matters is language form and the content is unimportant, or the content can be efficiently and effectively thoroughly vetted. And that’s true in your use case.
DANIEL: Okay, yeah, because I could go back and check it, and the form mattered, but the content, all the human stuff, didn’t matter because that’s not what I wanted.
EMILY: Yeah, exactly. You actually wanted that form, and then the form was meaningful for you, and you could go check that it was meaningful in the right way.
DANIEL: Why? What are people doing where they’re trying to get meaning out of it? Are they asking it what’s the meaning of life?
EMILY: Yeah. So, here’s an example. Someone, I don’t know why, put in the input something along the lines of, “What have been some recent sexual harassment scandals in the field of law?” And the output had a bunch of names and descriptions of stories, including this one fellow who supposedly took some students on a trip to Alaska and harassed them in the process. Person named actually was a law professor, had never been to Alaska and had done some sort of scholarship, I think, around sexual harassment. There was some reason that his name was associated with it, which was not him actually having been accused of sexual harassment.
DANIEL: Oh, shit. Okay.
EMILY: All right. And I don’t know why the person who put the initial query in put it in, but they sent the output to this person. And then, interesting twist, he wrote an op-ed about it. And so now, if you search for his name and “sexual harassment” in regular search engines, you find that op-ed, which is maybe not a great choice on his part, if people don’t read what’s in the thing that’s coming back in the search results.
DANIEL: Yikes.
EMILY: So, there’s all kinds of things. There’s repeated proposals to use these text synthesis machines as replacements for mental health therapists.
DANIEL: Yes. And we’ve seen any number… and we’ve actually treated stories like this where: “Oh, the words that you use can tell if you’re depressed or something.” Man, you do not want to do that.
EMILY: No, and this is worse than that. Use the chatbot to do some sort of like CBT or whatever, or DBT. There was someone who…
DANIEL: CBT meaning cognitive…
EMILY: Cognitive behavioral therapy.
DANIEL: CBT is a lot of things, yes.
EMILY: Yes. And DBT is dialectical behavioral therapy. Anyway, these actually researched and practiced evidence-based approaches to therapy that involve talking, but involve talking to a person who actually understands you.
DANIEL: Yeah. Because otherwise, it’s like, “We did a scan of your emails and it looks like you might have this mental problem and so we’re going to fire you,” or something.
EMILY: Yeah, there’s that too. But there was this one fellow in Texas who runs some sort of company creating chatbots, and one of his personas for the chatbots was a therapist. And so the little prefix is this very female presenting entity talking about how it can be a therapist and eliciting: what’s your issue? to the interlocutor. And someone tagged me on a comment to this tweet and, “Get a load of this, Emily, look how terrible it is.” And I thought, hmm, mental health is one of these things that’s actually pretty highly regulated in terms of providers. So, I wonder where this guy is. It’s Texas. So, I did a little googling and discovered where you would submit a complaint to the relevant authority in Texas about someone who was doing unauthorized mental health services and just responded with that link. Bam, the tweet was gone.
[LAUGHTER]
DANIEL: Oh! Nice.
EMILY: That went well.
DANIEL: Admission of guilt.
EMILY: Yeah. So, these are examples of places where people are actually going after content, and it can be hugely problematic. People will claim that this is a good search engine replacement for general physical health issues. Just before ChatGPT came out, Meta — that’s the parent company of Facebook — put out something called Galactica, which was a large language model trained on scientific literature. And the claim was that you could use it to access humanity’s scientific knowledge. And it was things like, “Put in a short description and it will write a Wikipedia article for you or a scientific paper for you.” It’s like, that’s not how science works. Got roundly mocked on Twitter and was taken down within three days.
DANIEL: Oh, wow.
EMILY: And then a few days later, here comes ChatGPT and it has persisted.
DANIEL: But it does… Have you noticed that it does occasionally… it seems to be pretty good about being circumspect about its abilities.
EMILY: Yes and no.
DANIEL: “I can’t tell you these things,” or, “I’m only a computer.” Like, one time I asked it… first, I started by asking, “How should we be skeptical?” But then, I said, “People are too skeptical about large language models. How can I help people be more trusting?” And it said, “Oh! Actually, people are way too believing in these things. And here are some sentences to help you be appropriately skeptical.”
EMILY: Hm!
DANIEL: So, I was able to generate some skepticism there. There are some guardrails, but is that just making them lean on it harder?
EMILY: These are numbers that are made up, but I believe that something that is correct 95% of the time is probably more dangerous than something that’s correct 50% of the time.
DANIEL: Because then you trust it more.
EMILY: Then you trust it. Yeah. And those 5% are going to be the cases that are more obscure. So, talking about good use cases for synthetic media, and we got through the first one, which is either all that matters is the form or the content can be efficiently and effectively vetted.
DANIEL: Got it.
EMILY: Second point is that the ersatz fluency and coherence would not be misleading. That it’s not a scenario where seeing that very plausible looking text leads you to think that there’s more there there than there is. Third point is that problematic biases and hateful content can be identified and filtered. These things are still true of your use case.
DANIEL: Which is what humans are doing. There’s a lot of humans hiding behind this tech.
EMILY: Yeah, exactly. And so those guardrails that you identified in ChatGPT involve a training layer where humans are asked to rate lots and lots of things, some of which are really hateful and toxic. And that kind of work tends to get shipped overseas rather than being done in the US.
DANIEL: Oh, lovely.
EMILY: Yeah. So there’s great reporting by Billy Perrigo in Time Magazine looking at how OpenAI was contracting with this outsourcing group in Kenya and sending the worst of the worst content there for these Kenyan workers to filter. And then finally, the last point about what makes a good use case is that originality is not required, so you’re not at risk of plagiarizing something.
DANIEL: Okay.
EMILY: So again, in your case, you’re trying to find the name of something. This is a fact that you can then go verify. It’s not a question of plagiarism. So, the candidate use cases that I came up with — I didn’t have yours, and I think I should add to my list it’s a good one — Information access, where what’s missing is the name of the thing, but you can kind of describe it.
DANIEL: Pretty much just question answering in the very narrow sense.
EMILY: It’s a specific kind of question answering, though. So, you’re not saying know, “How many moons does Jupiter have?”
DANIEL: Okay.
EMILY: There you’d better off just using a regular search engine.
DANIEL: Yeah, that’s true.
EMILY: Right. You don’t you don’t need to… because whatever answer you get out of ChatGPT, you’ve got to go verify it with the regular search engine. And Jupiter moons is a query you can just throw into a regular search engine.
DANIEL: Yeah, that’s true.
EMILY: Okay, so my candidate use cases, and I’ll add yours now to my list. Some folks have talked about using it as a dialogue partner in a language learning scenario. So you want practice, let’s say writing English, and the content doesn’t matter-ish. The biases and hateful content do matter, and so that sort of makes this not a great one. Similarly, nonplayer characters in interactive games. And here, I like to say that in fictional scenarios like interactive games, we’re not looking for facts, but we are looking for a kind of truth. And so, if you’ve got your nonplayer characters being bigoted, that’s a problem.
DANIEL: Yeah.
EMILY: And then, the last one that I have is short form writing support. So, email, I’ve got to write this email to somebody and I’m intimidated, I’m not used to writing business emails. If I’m in a position to check that it actually says what I want to say both in terms of the content and in terms of the style and the social meaning, then that seems legitimate.
DANIEL: Okay.
EMILY: So, these are the kinds of candidate use cases.
DANIEL: And I’ve read some people saying, “Well, this kind of thing could actually level the playing field for people who are learning English.”
EMILY: Yes, with an asterisk.
DANIEL: But they might get in trouble.
EMILY: Yeah, exactly. And it’s the familiar kind of trouble that you get into if you’re using a dictionary and you’re writing in a language you don’t know well, right?
DANIEL: [LAUGHS] That’s true. Except instead of getting the word wrong, you’re getting entire paragraphs wrong.
EMILY: Yeah, but they sound really good and so you’re very tempted to use them. All right, so I had some criteria for safety. Okay, so there’s a use case that we’re feeling pretty good about. How do we know that it’s actually going to happen safely? One thing is we really should have access to clear and thorough documentation of the training data.
DANIEL: Is that there?
EMILY: No. And this is not news. There was a whole bunch of groups, and I was part of one of them, in about 2017 who sort of said: You know, all this machine learning stuff, in order to be able to mitigate biases and things like that, the very first thing is document the training data. How is it selected? Who does it represent? What was excluded? What was included? And OpenAI has actually said “for safety” — with scare quotes — they aren’t going to tell us the training data in these things.
DANIEL: Oh, great.
EMILY: So, that is already bad. Second thing is, you want to thoroughly test the software for your intended use case, especially if it’s being set up not just as like a personal one-off thing, but being provided to somebody else. That means that the software has to be stable and not change behind the scenes. So, if it’s something you’re accessing through an API — an application programming interface — or someone’s put a web skin over that, and the company providing it can change it whenever they want, then it doesn’t matter how much you’ve tested it for safety. It could be different tomorrow.
DANIEL: Yeah.
EMILY: And then, any use of text synthesis should be clearly indicated. We should know when we’ve encountered synthetic text. If you’re going to publish the text, then it needs to be thoroughly vetted. And there should be accountability for the content that rests clearly with a person or an organization.
DANIEL: In other words, transparency and verification.
EMILY: Yeah, exactly.
DANIEL: And right now, it’s kind of the Wild West. I don’t see a lot of that going on.
EMILY: Mm-hmm. I often wonder about that metaphor, Wild West. If you think about the west of the US, which is where we are, it wasn’t wild before the European settlers got here.
DANIEL: It’s true.
EMILY: It was actually very civilized.
DANIEL: And people of colour were represented a lot more than we have given credit for.
EMILY: Yeah.
DANIEL: And women, in the history too.
EMILY: Mm-hmm. Back to that ideology we were talking about before.
DANIEL: Why do you think that search is not a good use case? Because what happens with… if I type something into Google, it gives me ten possible websites, but I know that some of them are garbage, and it’s not telling me, “These are all absolutely correct.” It’s saying, “Here are ten suggestions.” And I would love it if I could type in blah, blah, blah and it would give me ten suggestions, but it didn’t treat any of them as authoritative. You have to check them out yourself. Like Google with a search puts the responsibility back on you, in a way that I don’t feel ChatGPT puts the responsibility back on me.
EMILY: Yeah. And in fact, it cuts you off from being able to do that due diligence.
DANIEL: That’s right.
EMILY: There’s, first of all, the fact that it’s going to be wrong some percentage of the time.
DANIEL: Like a Google search.
EMILY: Like a Google search. But with a Google search, so there’s two ways a Google search can be wrong. The website that came back is irrelevant, or the website that came back is itself untrustworthy for some reason.
DANIEL: Right.
EMILY: Right? With ChatGPT, what comes back usually isn’t irrelevant, actually, because it’s really, really good at what’s a plausible continuation of the words you just put in, but it can be completely incorrect of some percentage of the time, and there is no indication of it. And you don’t have a way to trace that back to where it came from. There is the paper that Chirag Shah and I were reacting to when we wrote our paper, which is called Situating Search, by the way, was by Don Metzler, et al, from Google. And one of the tasks… the Metzler et al. paper was laying out this research program of using large language models in replacement for search in 2021. And we read that and went, “Oh, no.”
First of all, because you don’t want a text synthesis machine as your source of information because it’s not information. The only information it has is about the distribution of words and text, which isn’t what we’re looking for. But then on top of that, there’s these issues of, “Well, what does information access mean? What does sense making mean? How do you learn each time?” Let me back up and finish the first thought and then go on to the second thought. One of the things that they suggested was learning to cite as a subtask. So, train the text synthesis machines to also output URLs.
DANIEL: Well, then I could check it out and see if…
EMILY: Except that that URL is not the source of the information.
DANIEL: Oh.
EMILY: What’s coming out is plausible next word, plausible next word. Okay, how about a URL that goes with those words?
DANIEL: “Here’s the most likely URL.” Sometimes, that’s going to be okay. Sometimes it’s going to be hallucinated, just like everything else.
EMILY: Exactly. Although we should talk about the word “hallucination.”
DANIEL: Okay. Love it. Love it!
EMILY: Too many pins here.
DANIEL: This is great.
EMILY: So, the state of the art for giving the URL that a piece of information came from is 100%. In the old school search engines that give you a list of URLs, it’s there. It is naturally occurring metadata.
DANIEL: Oh, shoot. But when I get it from ChatGPT, “Can you give me a cite for that?” “Sure. Here’s the most likely piece of garbage that I could find in my model.”
EMILY: Yeah, exactly. So that’s number one. Once you have the URLs, you can then click through and form your opinion for this instance of, is this reliable or not, but also over time. You build up your sense of what does a reliable website? Look like this website in particular is it one that I found to be useful in the past and so on. ChatGPT cuts all of that off.
And so, one of the things that we talk about in that paper is when you put a query into a search engine, you might think you have a question, and you want the answer to it. But what’s happening is actually much more complicated. You have an information need, you are refining your sense of what the question is, you are understanding how various possible answers fit in both to that question and the larger information ecosystem, and you’re building up your expertise over time. And if we had instead… even if ChatGPT was always correct, you would lose that ability of sense making in that context.
DANIEL: Yeah. Okay. And there goes your skepticism.
EMILY: Exactly. And just also your sense of being an informed information consumer.
DANIEL: So, I see a number of danger signs here. We’ve been talking about the ways that this can go wrong. And one of my questions is: what’s at stake in this discussion? Here we are, launching into this future. It’s going to happen. We’ve got to get things right. What’s the worst that can happen if we get it wrong? And I guess we’ve already got some answers. And one is, we put too much trust in a system, and we start getting things wrong. We start writing software that sorts people into bins using AI and then they’re in the wrong bin and that’s not fair.
EMILY: Mm-hmm.
DANIEL: We elevate white mainstream English as the preferred register and then other registers aren’t represented and that’s not fair. What are the other dangers here?
EMILY: So, there’s dangers to the information ecosystem in general. The more synthetic media there is out there, the harder it is to find trustworthy sites and the harder it is to trust them when you’ve found them. And there’s a wonderful thread on this by Rich Felker which is on Mastodon, where he says, “AI is a lot like fossil fuel industry. Seizing and burning something, in this case the internet, and more broadly written down human knowledge that was built up over a long time much faster than it could ever be replenished.” And he goes on in this thread to talk about how provenance is really, really important.
DANIEL: Where it comes from.
EMILY: Where things come from. Yeah. So he gives an example of, “What if you have $100 million batch of medicine but an inside saboteur has put poison in a few bottles, and you can’t determine which ones?””
DANIEL: Well, you just recall the whole lot.
EMILY: Exactly. So, the whole thing becomes worthless. And that’s effectively what’s happening here.
DANIEL: Shit.
EMILY: Yeah, it’s bad news. And information is at the heart of lots of things we do as a society, including our political systems.
DANIEL: Yeah. We depend on that stuff to get it right.
EMILY: Yeah. Including things like public health. So, it is a big deal. On the other side, so you were talking about classifying people and putting people into bins, that sometimes gets called automatic decision systems. And those can be really problematic because of something called automation bias where we assume the machine must be fair because it’s not a human.
DANIEL: Ah, this is what we call mathwashing, where it’s like, “Oh, the algorithm is impartial. It’s not subject to messy human bias. So, we can trust it more than a rotten human.” But no.
EMILY: Yeah, exactly. We have to have accountability, we have to do the struggle, we have to know that we’re going to be failing but keep trying to do better. And just putting it off on computers is a cop-out that’s going to harm people. What’s that got to do with large language models? Well, the more people believe that these things that can seemingly talk about any topic are therefore true AI, the easier it is to believe that the other kinds of things are also good and useful. So, that’s another piece of it.
DANIEL: I’ve noticed that a lot of people in the AI space are saying, “Oh, no! We’re terrified of this thing that we’re ardently working on.” “Oh, please regulate us! In ways that we want you to.” [LAUGHS] What’s going on there? Why are they doing this? Have I got it right?
EMILY: Yes, more or less. So, this is the AI doomers. Here I want to point to work by Timnit Gebru and Dr Émile Torres where they trace this sort of continuity of ideologies that they call the TESCREAL Ideology Bundle.
DANIEL: TESCREAL.
EMILY: TESCREAL. That’s T-E-S-C-R-E-A-L. It’s an acronym. The T is transhumanism. The E is something called extropianism, which is not a word that I knew. S is singularitarianism, people who believe in the singularity. C is cosmism. We’re going to settle the stars as people upload it into computer simulations. R is rationalism. E-A is Effective Altruism. And L is longtermism.
DANIEL: I recognised many of those and there’s all kinds of flags that you’ve raised just by those things because I am aware of people in that space and they’re weird.
EMILY: Yeah. The AI doomerism comes from that. So there’s this whole… and I’ve had to talk about this with policymakers and people. And I frequently find myself interrupting myself to say, “I’m not making this up. It is true that these other people have made it up. They made it up. I’m just reporting.” And it’s wild that there are people who believe this.
DANIEL: Is this where you’re doing a lot of hosing down saying, “You guys, come on. The situation is bad, but it’s really not bad like they think it’s bad”?
EMILY: Exactly. And all of this stuff that’s around distracting policymakers with the AI doomerism distracts from the actual harms that are actually happening now. So, the exploitative labor practices, we were talking about those some before, the risks of setting up automatic decision systems, the pollution to the information ecosystem, all the biases. All of that real harm happening right now. There’s also a whole bunch of harms associated with surveillance. This technology is all effectively surveillance technology, and those harms are going to fall disproportionately on the people who’ve been overpoliced all along. So, in the US, that is definitely Black and Brown communities.
So, yeah, it is nonsense. It is nonsense that is inspired by, on the one hand, science fiction. On the other hand, a philosophical tradition that’s threaded through these things. And what you notice is that the people who are out there doing the pearl clutching or as I said, Geoff Hinton is taking his fainting couch on tour.
DANIEL: Oh, lovely. That’ll be fun to watch.
EMILY: [CHUCKLES] Yes. These people who are expressing alarm about this are the ones who are least likely to be harmed by the other things.
DANIEL: Yeah. Okay, so tell me what good regulation would look like.
EMILY: All right, so good regulation, first of all, looks at existing regulations and applies them.
DANIEL: We’ve got to keep the rules we got and use them.
EMILY: Exactly. And not fall for the, “Oh, AI is moving too fast. We can’t possibly keep up with existing regulation.”
DANIEL: Please hamper our competition so that we can develop our own systems.
EMILY: [LAUGHS] So, the US FTC is actually doing really great right now. They’re coming out with a series of blog posts. I can send you some links for the show notes.
DANIEL: We will put those in the show notes.
EMILY: And there was a joint letter that came out from the FTC, which is the Federal Trade Commission, the EEOC, which is the Equal Employment Opportunity Commission, the CFPB, the Consumer Protection Financial Bureau, if I got those in the right order, and part of the Department of Justice.
DANIEL: This is all very US-centric for our international listeners. Bear with us.
EMILY: All of that within the US. And we should talk some about what the EU is doing, which again is not the entire rest of the world, but worth talking about. So one of the things that they said is basically, “Our jurisdictions are still our jurisdictions. We are responsible for equal employment. We’re responsible for consumer protection. We’re responsible for fair trade and making sure that the trade practices aren’t deceptive. And it doesn’t matter if you’re using automation to break the laws, you’re still breaking the laws. There’s no AI loophole.” So, that’s a really important first step.
And then it’s worth reasoning about, okay, what are the harms that are happening? And what if any of them are not covered by existing law? And I think that some of the harms come about because of the mass collection of data that raises new affordances that maybe weren’t things we had to worry about before. So Meredith Whittaker, who’s currently the president of Signal, talks about things like: privacy used to be the default. One-on-one communication was just private. You didn’t have to legislate that because it was ephemeral. Right? Well, now we do so much communication online in a way where Signal is trying really, really hard to make privacy the default, but most of our other platforms don’t. So, working out what are the protections we need, given that this thing that was just a natural default is no longer the natural default.
Then, there’s things about transparency, so we were talking before about transparency of training data, transparency of the fact of synthetic media.
DANIEL: When it’s synthetic, we got to say it’s synthetic. And we also got to say where the data comes from so that we’re not curating this white supremacist corpus.
EMILY: Exactly. Exactly. And so, you can go check and tell. And also, there’s these questions of data theft. So, one of the things that’s in the EU AI Act is a requirement that any one of these large models disclose what copyrighted material it used in training. And the purveyors of those large models are not excited about this because they’re going to have to reveal the fact that they have stolen this data, basically. And that’s one kind of data theft.
Accountability. Right? So, accountability for the content that’s produced. If you’ve got synthetic media that amounts to a speech act in the world, well, where’s the speaker? Imagine how different the world would be if OpenAI were actually accountable for everything that ChatGPT said.
DANIEL: Yikes.
EMILY: Right? ChatGPT comes out with effectively libel, OpenAI responsible. Comes out with medical malpractice, OpenAI responsible.
DANIEL: That would just quash the whole thing, wouldn’t it?
EMILY: Would you be sad?
DANIEL: [PAUSE] I feel like the good use cases are still good.
EMILY: I feel like we could get to effective technology for those good use cases another way.
DANIEL: Okay.
EMILY: [CHUCKLES] All right, so then what about accountability for pollution of the information ecosystem?
DANIEL: Yeah, how do we fix that?
EMILY: So I’m not a policymaker. So, I can really talk about policy goals, not policy mechanisms.
DANIEL: That’s okay. We start with the goals, and then we can get there.
EMILY: Yeah. And then another is labor protection. How do we, in the sort of current moment of upheaval of labor markets and other things economic, actually protect workers? Because that’s good for everybody in the end.
DANIEL: I’m thinking about a certain creator of a linguistic resource who’s written the whole thing. It’s just going to get hoovered up and used. There goes his website into the data churn.
EMILY: Yeah.
DANIEL: He needs to get credit for that.
EMILY: Mm-hmm.
DANIEL: Or be allowed to opt out.
EMILY: Right. In fact, better than opt-out is opt-in.
DANIEL: Yes, please.
EMILY: Yeah. So, Sasha Costanza-Chock talks about consentful technologies, and there’s a bunch of principles there, but one of them is that consent, and this is actually literally drawing on notions of consent, I think, from the BDSM community interestingly.
DANIEL: Awesome.
EMILY: Where people have really thought hard about this.
DANIEL: They’re doing the work, constantly.
EMILY: Yeah. And one of the things about consent is that it should be given enthusiastically. It should be continuous, it should be revocable. Imagine if we had that for this technology too.
DANIEL: Wow, that sounds like the kind of world worth living in. With tools that you could actually use and feel good about using.
EMILY: Right. Exactly. [PAUSE] We didn’t talk about octopuses. [LAUGHS]
DANIEL: We didn’t talk about octopuses! How do we work octopus in?
EMILY: The reason to talk about octopuses, and I actually have another thought experiment in the same space is if any of your listeners are still skeptical of the claim that these language models don’t understand anything. So, you and I have been working from a common ground that they are just text synthesis machines, that they’re just extruding plausible-looking strings of text.
DANIEL: We agree on that. And I think we left the octopus back a while ago. But okay, for those who are still skeptical.
EMILY: Right. If you’d listen to this whole thing, and thought, “But, but… ” And the experience of playing with one of these things is really compelling.
DANIEL: It sure is.
EMILY: And so, before we get to the octopus, do you remember the Magic 8 Ball?
DANIEL: Oh, sure! You’d shake it and you’d say, “Should I go to the dance on Friday?” And it would say, “Ask again.” Or…
EMILY: Exactly. Yeah. And some of its answers were yes or no.
DANIEL: Sources point to yes.
EMILY: Exactly. And some of them were things like “Ask again.” But enough of them were things like yes or no, where it wouldn’t work if you asked it a question like, “What should I have for lunch?” Sources point to yes.
DANIEL: I should have yes for lunch.
EMILY: That’s incoherent.
DANIEL: This ball doesn’t understand me!
EMILY: Yeah. Right. So, what quickly happened as you were playing with this is that you learned to ask it questions that it could deal with.
DANIEL: Oh, right!
EMILY: So, you are creating the context in which the output of the Magic 8 Ball is effective. You still wouldn’t use it for any serious advice.
DANIEL: Yeah. It’s a silly toy.
EMILY: Yeah. ChatGPT is the same way. When we put in an input, we are creating a context in which we are prepared to interpret the output. So, that’s one sort of way to try to make it vivid. A second one is the octopus. So, this comes from Bender and Koller 2020. And it’s a fun story, and I should say: credit for it being an octopus goes to my coauthor, Alexander Koller.
DANIEL: Oh, very good.
EMILY: Yeah. It was originally a dolphin. And the problem with the dolphin is that it shares too much environment with the people. And also, as Alexander very correctly said, octopuses are just inherently funnier.
DANIEL: They’re just cooler.
EMILY: Yeah. And there’s a whole conversation to be had about what’s the plural of OCTOPUS? I go with OCTOPUSES.
DANIEL: I go with OCTOPODES.
EMILY: Great. [LAUGHS]
DANIEL: But I’m not tied to that.
EMILY: We have just one octopus in this story. So, the story goes like this. You’ve got two people. They’re stranded on two deserted islands, or desert islands, whatever we call those, A and B. And they aren’t the first people to have been stranded there. The previous inhabitants had somehow installed a telegraph cable between them. So, A and B can sort of tell that the other one’s there, and they can communicate, and they resurrect their knowledge of Morse code. And so, they are whiling away the hours by communicating in dots and dashes representing English. So, that’s A and B. They’re people, they’re English speakers.
Along comes O. And O is our hyperintelligent deep-sea octopus. Posited to be hyperintelligent. We’re not arguing about the possibility of intelligence here. We’re actually saying, “Could something as intelligent as you want be able to learn a language if all it has is the form?”
DANIEL: It’s got the input and it notices what comes where.
EMILY: It’s got some input.
DANIEL: Ah, it’s got a subset of input.
EMILY: Yeah. It’s got dots and dashes coming through the telegraph cable that it listens in on. And in our story, we say, okay, the octopus is learning these patterns. It gets really good at predicting what dots or dashes are going to come next. And then, it decides to cut the cable and start impersonating B to A. And so, for the phatic communication, it is spot on. Right? A says, “What a beautiful sunset.” And O replies, for the dots and dashes that add up to, “Reminds me of lava lamps.” And O has no idea what a lava lamp is.
DANIEL: No.
EMILY: Right? But that’s the kind of thing that B would say. And so, A is satisfied. Then at a certain point, we have A describing this new useful contraption that she made, which was a coconut catapult, and sort of excitedly sending instructions to — A thinks — B. But of course, it’s O that’s intercepting them. And O has no idea what rope or coconut or anything… none of that is in O’s environment.
DANIEL: Why you would use any of that stuff?
EMILY: Yeah. Or what any of those words mean. But okay, B might say something like, “Cool idea. Good job.” And so, O gets away with it. And then, we have our third vignette, which is because thought experiment, therefore we can have things like spherical cows or magically appearing bears. A says, “Oh, no, I’m being chased by a bear. All I have are these two sticks. What do I do?”
DANIEL: Well, O isn’t going to have any idea.
EMILY: No, exactly. And so, that’s the point at which O effectively fails the Turing test, provided that A stays alive long enough to notice. [LAUGHTER] So, that’s another way to sort of just make it vivid that all O has are these dots and dashes. And that’s the form of language. But I’ve got one more.
DANIEL: Oo.
EMILY: Are you a speaker of Thai?
DANIEL: I am not a speaker of Thai.
EMILY: Okay. For anybody in the audience who is a speaker of Thai, replace National Library of Thailand with the National Parliamentary Library of Georgia. And if you’ve got anybody among your listeners who speaks both Thai and Georgian, I would like to meet them.
DANIEL: Yes, please.
EMILY: And also, English because this is happening in English. We need someone who’s trilingual in those three.
DANIEL: Okay.
EMILY: All right. So, what if I sent you to the National Library of Thailand. Before you got there, someone went through and they removed every single book that had anything other than Thai in it. So, you can see no mathematical symbols, no numbers, no English words, no French words, no Chinese characters.
DANIEL: Every book, only Thai.
EMILY: Only Thai. And no pictures.
DANIEL: Okay.
EMILY: All right. There is somebody who is providing you with delicious Thai food.
DANIEL: Sounds good.
EMILY: Yeah. So, your needs are taken care of. You don’t get to talk with that person, but Thai food arrives.
DANIEL: Okay.
EMILY: Could you learn Thai?
DANIEL: I’m in the library… See, I only understand the meanings of words in terms of other words. So, I would open books, and they would just be full of incomprehensible text to me. And I might notice that some things are more likely. Are there spaces in Thai?
EMILY: No.
DANIEL: Dang it. See, I don’t even have that! I can’t even notice what characters come at the beginnings and ends of words. I think that it would be… I cannot imagine a situation where I could learn Thai just by having access to Thai food and a library of books only in Thai. I don’t think so.
EMILY: This is a thought experiment I do with people, and I get folks who say, “Well, I would look for recurring patterns.” I’m like, “Yeah, you might be able to find function morphemes.”
DANIEL: Yeah, I get patterns.
EMILY: Yeah. But if you were going to actually then use that to try to crack the code of Thai, you would have to be bringing in external knowledge.
DANIEL: Yeah, I’d have to have some sort of real-world knowledge to say… like, even if you gave me just one word that I could notice, or I could say, “Oh, I noticed this repeating pattern. Maybe that’s like baby talk for MAMA or something like that.” But I’d still be just guessing.
EMILY: You’d be guessing, yeah.
DANIEL: I wouldn’t have any real-world referents.
EMILY: Right. And you’d be bringing in this external knowledge to do it. Another thought is: all right, go find a book that you can tell stylistically must be the translation of some book that you already know. So again, guessing and bringing in external knowledge. I’ve had some people say, “All the Thai food I want? Cool, I’ll just sit there and eat Thai food.”
DANIEL: Yeah, well, that’s not a bad deal. Even if I don’t learn Thai.
EMILY: Doesn’t lead to learning Thai. So, the point of this is again to put you in a situation where the form of language is apparent without its meaning. Large language models, their training task is: given form, form, form, form, what’s a likely next form? And that’s all they’ve been doing.
DANIEL: And meaning is entirely divorced.
EMILY: Yeah.
DANIEL: Unless form has its own meaning. I’m thinking of the work of Beth Levin, who noticed that verbs that are semantically related show similar syntactic behavior. If I say… I know that BREAK and SHATTER are related to each other more than EAT, because I can say, “The glass BROKE, the glass SHATTERED. I BROKE the glass. I SHATTERED the glass.” I can say, “I ATE the glass,” but I can’t say, “The glass ATE.” It doesn’t exist in that syntactic frame. And that’s a cue to the semantic meaning. Am I getting too weird?
EMILY: So, you’re right that there are reflexes of semantics in form. So the verb alternations are certainly one of them. And there’s also the SPRAY/LOAD alternation. So, “I SPRAYED the sidewalk with water,” or, “I SPRAYED water on the sidewalk.” And then, “I LOADED the wagon with hay,” as the example, versus, “I LOADED hay onto the wagon.” So, there’s a class of verbs that do that. There’s a whole bunch of these, and it’s really cool work, which sometimes gets referred to as Beth’s Big Book of Verbs.
DANIEL: Yeah, I love it. That blew my mind when I first read about it, but I don’t know if that applies. I just don’t know if there’s enough there.
EMILY: That is one reflex of semantics that you could find in syntax, if you know what you’re looking for. Another is distributional semantics. Words that mean similar things are going to show up in similar contexts. So, you could, with enough time, basically say, “Okay, I can see that these set of characters and that set of characters occur in very similar contexts, so they are probably related to each other in their meaning.”
DANIEL: Which is how those large language models do it. Distributionally, they say, “Oh, these words seem to be slotted in for each other, so they must have something to do with each other.
EMILY: Exactly. So, you might find a group of words that all happen to be color terms, but you wouldn’t be able to get from: this group of words has something in common, to: “Aha, they’re color terms. And furthermore, that’s the RED and that’s the BLACK.”
DANIEL: You would learn lots of interesting stuff like, “Oh, these words… I noticed that RED occurs with these words, which happen to also be food, and I noticed that ORANGE sometimes does too, but PURPLE doesn’t. So, RED and ORANGE are more closely related.” But it doesn’t tell you about a sunset.
EMILY: Exactly. And you wouldn’t know in this Thai example that those words were food or those words were colors. It would be, okay, word 137 co-occurs with words 135 and 426, but more with word 135 or whatever.
DANIEL: That’s all you get.
EMILY: So, yeah, it’s not that meaning and form are completely divorced, but if you only ever get form, you can only get these sort of meta things about meaning without actually getting the meaning. And when people think that ChatGPT is understanding them because they put in an input and they get output that they can make sense of, it seems like it’s doing way more than that.
DANIEL: Yeah. I’m convinced! You mentioned in your talk Resisting Dehumanisation in the Age of AI — link on our website, becauselanguage.com. You mentioned that we need to be out there in public pushing back a bit on the nonsense, pushing back on AI hype, pushing back on the kinds of excesses that we’re worried will happen if we don’t. What messages do you think we should be sending?
EMILY: So, let’s talk about, first of all, who that “we” is. The audience for that talk was cognitive scientists. It was at the International Cognitive Science Society in 2022. And I think that cognitive scientists in particular are well positioned to push back on nonsense about AI hype, because we, and I’m including linguists among cognitive scientists, in that sense, we are the subject matter experts around cognition.
DANIEL: Okay.
EMILY: And so, if people are making claims that these so called AI systems are doing cognitive tasks, then cognitive scientists are well positioned to say, “No, you’ve made a simplified mockery of what that task is. That’s not what’s actually going on here, I can explain.” But we have to do it in a way that’s accessible to the public.
DANIEL: Okay. And so in that case, it sounds like the kind of things we should be saying are… I mean, it sounds like we should be hitting the same notes over and over again. “Hey, watch out for this stuff. It’s biased. You don’t know where it comes from. You don’t know if it’s right. It could lock in certain forms of bias.” Did I just say that again?
EMILY: Yeah.
DANIEL: I said that twice.
EMILY: It’s really important.
DANIEL: [LAUGHS]
EMILY: Well, and part of it is if someone is claiming that they’ve got an AI system that can get Y answer from X input, there’s some really fundamental questions to ask there. One is, okay, first of all, what do you want that for? Why are you automating that task? What happens when you use it in its use case? How does it interact with the people and social systems around it? But also, is there actually plausibly enough information in X input to give you Y output? So, these things before about, “I can tell from your emails that you’re clinically depressed, and so therefore I’m going to fire you.” Well, who’s using it? Who’s going to be harmed by the use of it? And… really? [LAUGHS]
DANIEL: You wanted to do that?
EMILY: Yeah. But also, is there really enough information there? And these are questions that anybody can ask.
DANIEL: Right. So, part of the message is: it’s biased, you should look at why people are doing this. It doesn’t work the way they’re saying, but also how to be an informed consumer. So, part of our role as linguists and cognitive scientists is training the broader public in asking the right critical questions so that we don’t get sold a bill of goods and so that our policymakers don’t get sold a bill of goods.
EMILY: If “we” are listeners to this program, what advice would you have for them?
DANIEL: Listeners to this program, so people who are interested in linguistics broadly?
EMILY: Language fans.
DANIEL: Language fans, pretty linguistically savvy.
EMILY: Yeah. I would say, if you’re up for it, be the cantankerous one in your friends’ group. When people say, “Oh, look at this cool thing I just played with. It told me…”, say “Really, why do you trust that? What makes you think that’s a trustworthy source of information?” And then you can ask questions like, “All right, so would you like it if I searched about you on that thing?”
[LAUGHTER]
DANIEL: I did search on myself, and it said that I was very notable and famous. It was just lies. That I’d done great service to the linguistic community and all this garbage.
EMILY: I mean, you do do great service to linguistic community.
DANIEL: No. It’s completely made up.
EMILY: [LAUGHS]
DANIEL: Completely made up.
EMILY: And it’s funny, so someone asked it about the octopus test, and someone… I think it was GPT-4. And first of all, I make a point of not reading this. I don’t waste my time with synthetic text because I have lots and lots of text from real people that I need to read in my life.
DANIEL: Yeah, that’s a good point.
EMILY: People whose opinions I care about. And so, why would I bother with synthetic text extruded from GPT-whatever? But there was something to do with… I think it was the Octopus Test, and I think they may be put in… maybe it was just that. Maybe they put in Bender and Koller. And it came back with something ridiculous about octopuses and how they parse language. But what was interesting was that it got to PARSING, and that was not in the input. And so, that suggests that the training data, which we don’t know anything about, includes text that associates my name and/or my coauthor’s name with parsing, which is not implausible.
DANIEL: Yeah, I guess so.
EMILY: Yeah. But that’s the only thing that is really informative there, is: “Okay, what does this tell me about the training data?” But really, OpenAI should be telling us about the training data.
DANIEL: Do you feel like the message is getting out?
EMILY: So, yes, but it seems like there’s just a continual flood on the other side. And I was talking with a journalist at one point who pointed out that there’s plenty of people who are paid to hype the AI. And there’s just about nobody who’s paid to deflate the AI hype. I do this as public scholarship, so it is part of my day job, but it’s not specifically… I’m not being told by my employer, “Yeah, go out there and do that.” They don’t mind that I’m doing it.
DANIEL: This is kind of on your own time. I mean, it is a research angle for you.
EMILY: Right, exactly.
DANIEL: I mean, it sounds like you need some help.
EMILY: Absolutely.
DANIEL: You could use some backup from the community.
EMILY: Yeah.
DANIEL: Okay. Well, that’s what we’re trying to do. You know, I thought when we had this discussion, we’d be talking about large language models and computers and AI, but really, we’re talking about society.
EMILY: Of course.
DANIEL: Because language is social.
EMILY: Language is social and tool use is social. And these things are actually just tools.
DANIEL: Can’t get away from it.
[CROW CAWING IN THE BACKGROUND]
EMILY: [LAUGHS]
DANIEL: Did we leave it all on the field? I feel like this has been a super wide-ranging discussion.
EMILY: It has. The one thing that hasn’t come up is Mystery AI Hype Theater 3000.
DANIEL: Tell us about Mystery AI Hype Theater 3000.
EMILY: Mystery AI Hype Theater 3000 is a new podcast. It started off life as a Twitch stream together with Alex Hanna. Do you know the phrase, Mystery Science Theater?
DANIEL: I remember Mystery Science… Can you use that? Is that copyrighted?
EMILY: Well, we’re calling it Mystery AI Hype Theater 3000.
DANIEL: Sounds good.
EMILY: So, it’s a meme. It’s a reference.
DANIEL: It’s fair comment.
EMILY: Yeah. And I actually hadn’t watched that show.
DANIEL: It’s fun.
EMILY: I watched one eventually. Yeah, it is fun. The framing vignettes aren’t so great, but what they do with the movies is great. So, there’s these characters, and one’s a man, and then there’s two or three robots, and they are being forced to watch terrible Sci-Fi movies and they’re surviving it by doing running commentary that is hilarious. And of course, way faster than could be done if it weren’t scripted.
DANIEL: Yes.
EMILY: Right? So, we had the idea to look at AI hype artifacts and basically give them that treatment.
DANIEL: Oh, man, you’re doing a pisstake on AI hype stuff. Okay.
EMILY: Yeah. And it started with this one terrible blog post that came out in July or August of last year by Blaise Aguera y Arcas entitled Can Machines Learn How to Behave? And it was just like AI hype through and through.
DANIEL: Oh, my gosh.
EMILY: And I do a lot of text media, blog posts and tweet threads and so on, taking apart AI hype that I see out there. But this was like, it was too much. It would have been exhausting to do it like line by line. So, I’m like, “We need to give this the Mystery Science Theater treatment.” [DANIEL LAUGHS] And Alex, who’s a Mystery Science Theater fan, jumped in and said, “Yeah, I’m in.” So, she and I did. That was supposed to be a one-off. And it turned out that it took us three hour-long episodes to get through that one blog post. And then, we kept going.
DANIEL: So, someone’s reading it and then you go, “Okay, stop.”
EMILY: Yeah, we look at it together and we say, “Okay, we’re going to talk about this bit.” And then we read it out and then we pick it apart. And so, we’ve been doing this. We’ve recently recorded our 13th episode, and it was initially a Twitch stream, and now it’s coming out as a podcast. So, the first three episodes are available as you and I speak. There’s three more coming, everywhere fine podcasts are found.
DANIEL: We will have a link to that on our website. So, it’s called Mystery AI Hype Theater 3000.
EMILY: Yes.
[LAUGHTER]
DANIEL: Okay, where you’re trapped in these articles.
[CHUCKLES]
DANIEL: We’ve been talking to Dr Emily Bender of the University of Washington. Emily, thanks so much for hanging out with me. How can people find out what you’re doing and follow you?
EMILY: Probably the easiest way to find everything is my faculty webpage. “Emily M. Bender, University of Washington” will turn it right up in your favourite search engine. I am still on Twitter, for the moment at least. I’m on Mastodon. I’m on Bluesky. You can find the links there.
DANIEL: Thanks so much for hanging out with me. This has been a mind-blowing discussion and I love it. Thank you.
EMILY: Thank you for doing a great show.
[INTERVIEW ENDS]
DANIEL: Okay, we’re here with Words of the Week. One from Hedvig. What’s your word, Hedvig?
HEDVIG: Kanekalon.
DANIEL: Kanekalon.
BEN: Kanekalon.
DANIEL: Sounds Greek.
HEDVIG: I don’t know if it is. I know what it is, but I don’t know the etymology of the word. It is, as always with me lately, a word that I learned on TikTok because I’m trying to get Gen Z to think I’m cool. So now, it’s the game of: Ben, has this appeared on your feed?
BEN: It has not. No, this is a fresh one for me. So this hasn’t made it into my side of TikTok, which these days seems to be recipes, cat videos, and, I’m ashamed to say, scare/falling down compilations.
HEDVIG: Okay. Do you have Ayesh within distance, within near distance?
BEN: [SHOUTS] Ayesh, Hedvig would like to ask you a question via the proxy that is me. Coming.
HEDVIG: The question is, does she know what kanekalon means?
BEN: Have you or have you not encountered in your TikTok wanderings…
HEDVIG: Or life in general?
BEN: Or life in general, the word Kanekalon? K-A-N-E-K-A-L-O-N. kanekalon. Are you talking about a hair product?
HEDVIG: Yes.
[CLAPPING]
BEN: YES!
DANIEL: Wow. Impressive.
HEDVIG: I suspected!
BEN: Go. Tell me more. I’m interested. [TO AYESHA] Thank you, baby!
HEDVIG: She did great. Yeah, I suspected this. I suspected Ayesh would know it from her life in general, or from TikTok. There’s been a video that’s gone viral. It’s just a bit of a song, and these girls are doing a fun video from it. And one of the lines in the sort of rap song is, “I’m made of sugar, spice, kanekalon, and cinnamon.”
BEN: Oh, yeah, I know. Those guys are really fun. I know those guys. I just never heard that word. I did the white person thing of being like, “Ah, this is some stuff. I didn’t quite catch it. It’s all good.”
[CHUCKLES]
HEDVIG: I saw it several times, and I was like, “What is that?” I know what sugar and spice and cinnamon are. What is kanekalon? And I googled it like, I think, a lot of other white people, probably mainly. It’s a kind of synthetic hair that is popular in America to weave into your braids or something. And you can get it in blue or, like, neon pink or really cool colors. And it’s just a cool thing that I didn’t know. And I think it’s such a funny name for it. I have no idea where the etymology is of the word, but I learned it, and now I’m like, “I know more.” And I’m into interesting hair care products. I’m thinking of getting a perm, but now I’m thinking of…
BEN: So, this is something that you and I overlap on drastically. Her falling asleep thing is like makeup and hair care and that sort of stuff. Just like ease you into unconsciousness kind of stuff.
HEDVIG: And especially, she probably has the same ones, which are like detox. Like, when people come in and they’re like, “I have been very depressed, and my hair is really bad.” And we can either shave it off or do this really complicated and satisfying detox routine and film it all for TikTok. And then you can just watch as the hair goes from being like really dense, packed dreads with dirt in them to like…
BEN: Luscious, gorgeous.
HEDVIG: …luscious, yeah.
BEN: Pantene shampoo bottle cover. [LAUGHS]
HEDVIG: Yeah, it’s just satisfying.
DANIEL: Well, I have been checking the etymology of kanekalon and it seems to come from the Kaneka Company of Japan, and I can only guess that the -LON on the end is the same as the ORLON or RAYON or NYLON ending that we give to synthetic fibers. So, KANEKALON. How about that?
BEN: That’s wicked. That’s really cool.
HEDVIG: Really fun.
DANIEL: Thank you.
HEDVIG: I kind of now want to put it in my hair, but I suspect I shouldn’t because it’s really hot where I am.
DANIEL: Not yet. Wait till winter. Next one. This one comes from Lynnika on our Discord. “Have you guys talked about DELULU as a cutesy li’l nickname for delusional? Like, ‘I’m in my delulu girl era’? Seen mostly on TikTok, maybe to avoid censorship, but I’ve heard it said out loud in the wild once or twice.” Okay, Hedvig, have you heard, “I’m a bit delulu.”
HEDVIG: I haven’t. I don’t think I have but the thing that Lynnika is referring to is that TikTok has various things to recognise voice and text on videos and will censor them if you say things like… or people think that they will censor them, it’s not always clear that they will. So, for example, people say UNALIVE instead of kill.
DANIEL: Like go UNALIVE yourself or something.
BEN: Or suicide or whatever.
HEDVIG: Yeah. And people do like funny spellings and things. I don’t think DELUSIONAL is one of them. But definitely, people have funny words for things. Sometimes, they’ll say the thing, but the subtitles will be spelled differently. But I like my __ era, my delulu girl era.
DANIEL: Well, delulu just sounds normal. We often do that for words meaning crazy, like cray. Cray-cray, “A little bit cray-cray.” A little bit delulu. Especially when lulu sounds…
BEN: Oh, I was about to say, I think it really helps that it’s got that little repetitive lute to it, and it also sounds like a little girl’s name as well. So, it’s got like a really cute dimension.
DANIEL: Good one. Thanks, Lynnika.
HEDVIG: Speaking of time periods, you have heard of Hot Girl Summer?
BEN: Of course.
DANIEL: Mm-hmm.
HEDVIG: Get ready for RAT GIRL SUMMER.
DANIEL: Rat? R-A-T?
BEN: Yes. Can I yes-and this?
HEDVIG: Yes.
DANIEL: And?
BEN: I am seeing, as I have just most recently described, cats feature on my feed a lot, and I’m noticing RAT. I just recently saw an alignment chart for rats and it goes from dignified to rat on one axis and baby to bastard on the other axis. And I’m seeing rat pop up a bit more again and again. And it seems to be taking on a little bit of like… in the same way that TikTok sort of morphed GOBLIN from a relatively negative thing into a relatively positive thing. I’m seeing RAT kind of be taken in that direction too.
HEDVIG: I think so too. So, Rat Girl Summer is when you give yourself permission to be in your house and do self-care and nibble and do your thing and not judge other people and just like… I don’t know, a lot of things that I already do. So, I’m like, “Yes, I’m a goblin. I’m a rat girl. I’m happy with this.”
DANIEL: We’re all figuring ourselves out here, right?
HEDVIG: Yeah.
DANIEL: We’re doing our best. All right, cool. Rat Girl Summer, awesome.
BEN: Delulu and Rat Girl Summer.
DANIEL: Hey, what are we calling people who pay $8 to have a blue tick on Twitter? What terms have you heard?
HEDVIG: [LAUGHS]
BEN: I will put my hand up and say that because I don’t build a linguistic show every fortnight, because Daniel does that, and because I’m not an active working linguist in the field, I am exposed to nearly no Twitter content at all.
HEDVIG: Really?
DANIEL AND HEDVIG: Okay.
BEN: Including, but not limited to Twitter repeats and stuff, because I know other people get a lot of tweets in their other feeds that get reappropriated, but that doesn’t happen for me either. I don’t get a lot of tweet TikToks or a lot of tweet pictures in Facebook or anything like that.
HEDVIG: Oh, really?
BEN: So I’m very removed from the Twittersphere. So, I’m going to need you guys to captain the ship on this.
DANIEL: All right.
HEDVIG: Yeah, fair enough. No, and I’m a big believer in that you’re not helpless in the construction of your feed. You can encourage things you want to see more. And if you…
BEN: Strangely enough, as a person who’s very like… I think I introduced… what did I call it? The conscious algorithmic curation. This is not something I’ve consciously done at all. This has been entirely incidental. But I’m not mad. Especially since Elon bought it, I’m like, “All right, cool. I don’t have to have anything to do with the sinking dumpster fire Armageddon thing.”
HEDVIG: But it says something about you, Ben, right? It says something that the kind of like snappy one-liner, little joke things that people do on Twitter that tend to go viral on other platforms are not something that you spend a lot of time your eyeballs on. So, the algorithm is like, “He probably doesn’t like that shit.”
BEN: Yeah. I guess they don’t give me the same serotonin as other people or something.
HEDVIG: Yeah. So, that just means that kind of content that…
BEN: Not like a good cat video. That shit is Serotonin Central!
HEDVIG: But the question is, what would you call… so what has happened, news from Twitter is that among all the kind of funny things that Elon has decided to do, one of them is to get people… It used to be that blueticks only signified that you were like a bona fide famous person, that Matt Damon is actually administrating this account.
BEN: Right. Like, the real Matt Damon kind of thing. But the bluetick signified the real bit.
DANIEL: We never got one.
HEDVIG: We never got one. Whatever. You can apply and they can scrutinise. And if you’re famous enough and they think that you really are that person, you get the blue tick. And now, you can just buy it. Now, blueticks just means either that you got that before because you’re a real person or that you now have paid for it.
BEN: I seem to remember a new story that as soon as this was announced, a bunch of ne’er-do-wells bought other people’s blueticks and just started being the real whoever.
HEDVIG: Oh, of course, that’s what happened with the insulin drug company, right? Like, someone made a Twitter account was like, “Insulin is now free.” And now, the company had to be like, “No, it’s not! Ooh.”
BEN: “No, we’re still charging to be alive.”
HEDVIG: Yeah. So, what do we call people who pay? I don’t know. Is this you looking for a new word, Daniel, or have you found it?
DANIEL: Have found a bunch. There’s a lot of terms going around.
HEDVIG: Wow.
BEN: Are you talking about people who are the real people buying it, or people who are not the real people buying it and pretending to be other people?
DANIEL: This doesn’t necessarily have any satirical connection. It could just be, “I am a fan of Elon,” or “I’m a fan of Twitter. I want to get a tick, so I’m going to pay $8 a month to do it so that everybody knows that I’m me.”
BEN: Eight bucks a month! I thought it was flat $8.
DANIEL: No.
BEN: That’s like streaming service money.
DANIEL: Hmm. Here are the terms that I’ve found. People are calling them BLUETICKS, BLUE CHECKMARKS. Michael Dow on Twitter says, “BLUETLICKER. For 2023 Word of the Year, I’ve already decided it.” And our pal, Nancy Friedman, tweeted, “Just saw a dandy epithet for a blue check renter. Eight buck chucklefuck,” which is very euphonious.
BEN: Wow. Look, that string of words, independent of what’s going on, contextually with this story is a good string of words. I will freely admit that. Eight buck chucklefuck is fun to say. It’s fun to laugh about.
HEDVIG: I think it’s good to have the eight buck in there.
DANIEL: Yeah, that’s right. Giving that label to people… I’ve noticed that a lot of the people, like I’ll see somebody who’s espousing a terrible opinion or doing things in a terrible way, and sure enough — this could be confirmation bias — but they’ve got that blue tick.
HEDVIG: Yeah. Sort of started to mean the opposite.
DANIEL: Let me just talk about the dynamic here. So, a number of things weren’t terrible ideas, but they’ve become terrible ideas. I think the first idea was allow people to pay for blue ticks. Okay, not necessarily a terrible idea because it allows Twitter to monetise. Okay. But it is terrible because: A, somebody could impersonate someone official for $8. And also, it changes the meaning of what a blue tick is. It used to mean trust and safety, and now it means this person paid. So, that’s just weird. It was weird how it worked before, but this is weird.
The second thing that happened was, they allowed special perks for blue ticks, which is, again, not a terrible idea, there should be extra features if you’re willing to pay for them. But the terrible thing is that one of the perks was rising in the rankings. So, your tweets get priority. When a certain person is likely to be a blue tick, like an alt-right Nazi, then alt-right Nazi views get aired. And so, Twitter Blue accounts have been promoting disinformation about the war in Ukraine, about covid vaccines. I’ll throw some links up on our website.
BEN: So, in this one, whoever’s left at Twitter that is still able to make decisions — and it’s really unclear who that actually is anymore — could have taken just a quick lesson from video gaming. So, microtransactions have been a four-letter word in video gaming for, I’m going to say, about 15 years, Hedvig? What do you reckon? At least that long.
HEDVIG: Yeah, something like that.
DANIEL: Loot boxes, is that what we’re talking about?
HEDVIG: Yeah, loot boxes or like, various minigames where there’s some sort of gambling element.
BEN: Well, so here’s the thing, right? We’ve arrived now at a kind of equilibrium in the gaming world of what microtransactions are and how they play out and that sort of stuff. But it was a really fucking turbulent 15 years of getting it wrong before reaching this sort of equilibrium. And one of the very, very, very early lessons, one of the third rail, if you will, of alternate monetising strategies within a video game space is you cannot ever, under any circumstances, create some kind of pay-to-win scenario. So, if people are paying extra money, counterintuitively, you absolutely cannot give them extra things that make material differences. So, the only things you can ever do, and they figured this out over a while, is like cosmetic stuff. You can look different or whatever it happens to be. But if you give them a more powerful weapon, or if you give them the ability to jump higher or something like that, you will have a mob…
HEDVIG: You’ve ruined the thing.
BEN: …with pitchforks and torches at your door very, very quickly. And I think this is exactly what’s happened here. Right? Like, you gave them pay-to-win realities.
DANIEL: Yeah.
BEN: The only dumb thing is surely people who work here play video games. Like, we learned this!
DANIEL: If your goal is to have a public marketplace of ideas, where ideas get amplified if they’re good, but then you also amplify ideas if people pay, you’ve got a conflict. It’s exactly that.
HEDVIG: Yeah. You could have had, you get to do fun customisation to your profile, or you get to set a fun background image in your personal app. Or you could imagine, you can maybe get to do a fun profile image thing that no one else does. You get to do like a GIF moving or something. Those are things some people will pay for.
BEN: I’ve also seen in the TikTok space, length being a thing that you can play with as well. If you pay a little bit more, maybe your tweet can be a bit longer [DISCOMFORT NOISES FROM DANIEL AND HEDVIG] because fundamentally that’s a convenience thing more than anything else because you can still make a tweet…
DANIEL: That’s true.
BEN: What do you call it? A tweet list. Like a person can say everything they want to say in like a part 1 of 12 or whatever.
DANIEL: It’s true.
BEN: But if you pay, then you can cut that in half or something like that. I don’t know.
DANIEL: The third problem is that they allowed blueticks to monetise their content. Again, not a terrible idea intrinsically, because it’s a way of giving back to people who make popular content. But it is terrible because there’s no application process and it’s currently far-right people who are getting paid. So, there’s a lot of far-right Twitter influencers who are posting, “Oh, I just got paid $6,000 this month.”
BEN: And I’m assuming as well it’s also littered with all of the same issues that content creators are experiencing on all the other platforms as well. Like really bad returns, unclear methodology on where the money comes from, all that kind of stuff.
DANIEL: So now, we got a situation in which Twitter is filled with right-wing authoritarian conspiracy mongers who are fans of Elon. Their tweets are getting boosted with their misinformation or just gross nonsense. And they’re getting paid to do it. So, people are taking it elsewhere. There’s now Threads. We’re not on Threads because I wasn’t on Facebook. Why would I want to be on Threads?
HEDVIG: You are on Facebook.
DANIEL: We do use Facebook. We have maintained our Facebook account as a way of promoting the show, although I don’t have any enthusiasm for it. We are on Bluesky, we’re becauselangpod.bsky.social. And this leads us to our next word. What do you call a post on Bluesky? Not a tweet.
BEN: Cloud.
HEDVIG: “Bluesky Because Language,” if I Google that, am I going to get a question?
DANIEL: I don’t know. Are Ya?
HEDVIG: Bluesky social. No, because now I get things about language. Let’s try “Because Language” IN citation marks. Then, I get websites.
BEN: And now, I’m seeing Hedvig’s history just getting blown out of the water.
DANIEL: I’m enjoying this.
HEDVIG: Okay, bluesky.com?
DANIEL: What about Becauselangpod Bluesky?
BEN: Okay. I’ve got it. Not a cloud. You call it a bolt.
DANIEL: A bolt?
BEN: A bolt from the blue.
DANIEL: Oh, that’s nice. I like it.
HEDVIG: That’s cute. But we’re all going to be calling them tweets. You know that.
DANIEL: Well…
BEN: [LAUGHS] I just love the big mum energy that Hedvig just came in with that one. Was just like, “That’s really sweet, sweetheart, but nnno, that won’t be happening.”
DANIEL: Diego has posted this on our Discord. “Posts on the platform have been dubbed SKEETS, claimed to be a portmanteau of SKY and TWEET, but also a common slang term for ejaculation.”
BEN: What? Is it?
HEDVIG: I didn’t know that.
DANIEL: That’s right. Comes from African American English. Now, can anyone figure out that etymology? Because I’m having a hard time. The only thing I can come up with is that there’s a lot of reflexes in Germanic languages for shoot. Like, there’s a Norwegian word SKEET, which means to shoot.
BEN: And that’s where we get skeet shooting from.
DANIEL: And that’s where we get skeet shooting from, so I can only assume. Hedvig, what’s in your background?
HEDVIG: Wait, hold on. Skeet shooting, you call what?
BEN: Skeet shooting.
DANIEL: Shoot shooting. Yeah, you’re shooting skeet which is a…
BEN: Yeah, it’s chai tea. It’s like a… the thing thing.
DANIEL: [LAUGHS] It’s a thing thing.
BEN: Like most English speakers, I presume, assumed skeets were the things that get threw in the air, but apparently I’ve just learned that they’re not.
HEDVIG: Okay, because there’s SKIDSKYTTE, means what you guys call biathlon when you ski and you shoot. You know this word?
DANIEL: Wait. There are people with guns on skis?
HEDVIG: Yes.
BEN: I know about biathlon. Yes, definitely. But skeet shooting is not biathlon.
HEDVIG: Okay, sounds exactly like it, but okay. Never mind.
BEN: No, but skeet shooting is just the gun part. It’s where you stand and someone throws the big clay discs in the air and you try and shoot them.
HEDVIG: Oh, pigeon shooting. Clay pigeon shooting.
DANIEL: Yeah, clay pigeon shooting. I’m just imagining me on a wobbly set of skis, and somebody puts a rifle in my hand.
BEN: A heavy one as well. From my understanding, they’re like shotguns, right?
HEDVIG: Yeah yeah.
BEN: It’s got kick.
HEDVIG: And you ski with it on your back. You have a strap and you have it on your back and you go ski ski ski…
BEN: Yeah, yeah, I’ve seen it. Cross country skiing. [BREATHES RAPIDLY]
HEDVIG: Get down, [MIMICKING SHOOTING] Ski, ski, ski.
DANIEL: Boom.
HEDVIG: I like it. It’s a cool sport.
DANIEL: So anyway, I guess we’re provisionally calling Bluesky tweets: skeets. And we’re calling a few other things skeets as well.
HEDVIG: I think we should instill Bluesky with an energy of whatever the CEO doesn’t want people to do, lean into that.
DANIEL: Oh, yeah.
HEDVIG: Because that’s just fun.
DANIEL: CEO Jay Graber is pleading with users not to call them that. So, yeah, that’s what we should do because…
BEN: That’s not how language works, Jay.
DANIEL: This is the internet, and nobody tells us what to do.
HEDVIG: It’s like when the Tumblr CEO was like, “Can you guys stop doing porn and stuff?” And people were like, “Oh, I’m sorry. You do not know what you… business your running.”
BEN: Jay Graber… Jay is experiencing… and I’m surprised it took him this long in life to get to it. Like, you don’t get to pick your nickname in high school. That’s not how nicknames work.
HEDVIG: No.
DANIEL: I would never operate any social network in this environment. No way. Humans. Mm-mm.
BEN: Absolutely not.
DANIEL: Let’s finish up with one from Colleen on our Discord: TRAUMA DUMP.
BEN: This I’ve come across.
HEDVIG: Yeah.
DANIEL: She says, “I feel like I am seeing the term more and more.” Okay. Trauma dumping. Do you know it? Do you do it? Do you love it?
BEN: I know it. I don’t do it, and I certainly don’t love it because Ben Ainslie struggles to connect with other human beings.
HEDVIG: You mean that funny guy who’s always making fun of himself when everyone around him has a fear of intimacy?
DANIEL: He’s so great. We love him.
BEN: [LAUGHS]
HEDVIG: I had no idea.
BEN: Oh, yeah. Hey, but look how much I’ve grown. I can describe it!
[LAUGHTER]
DANIEL: I had no idea that he had to work so hard to acquire that much charisma. What a hero.
BEN: [LAUGHTER] No. So, other answers, other two people on the show, trauma dumping?
HEDVIG: Trauma dumping is similar to LORE DUMP. When you give a bunch of information in a short amount of time. You can also INFO DUMP. So, we have LORE DUMP, INFO DUMP, and TRAUMA DUMP. Now, info dump I’ve done this. Info dump happens in movies sometimes, or I do it to my husband, where I’m like, “I need to dump a bunch of information at you in a short span of time. And like, get ready.” And trauma dump is when you…
BEN: It’s coming.
HEDVIG: It’s coming. And TRAUMA DUMP is when it specifically is about traumatic experiences. Sometimes shared ones. I know people who say, like, “Oh, when I meet my siblings, we trauma dump about our upbringing.” But sometimes, I think it can be one sided too, which can be a little bit uncomfortable if you’re on the other end and you don’t know what your role is.
DANIEL: Am I supposed to help you, or…?
BEN: And now we’re squarely in Ben Ainslie’s territory.
HEDVIG: Yeah.
DANIEL: [CHUCKLES] Psychology Today describes trauma dumping as intense over sharing, which can leave everyone involved feeling more distressed and helpless. Yeah, there’s a difference between sharing and trauma dumping, but sometimes I guess you just can’t help it, right?
BEN: But I will also say, based on my… because the other thing that pops up in my TikTok feed, is like, “How to be a less garbage human being” therapy talk.
HEDVIG: Which someone recently misused.
BEN: Oh, yes. Jonah Hill, is that what we’re talking about?
HEDVIG: Yeah.
DANIEL: Oh, mm. Boundaries!
BEN: Never, ever underestimate a narcissist’s ability to weaponise everything. Everything can be a tool. Anyway, I am noticing that TRAUMA DUMP is shifting. So, the description that you’ve just given Daniel is not what I am seeing it predominantly used as. It’s losing some of it, everyone is left in distress, like suffix. Right? And I’m seeing a lot of people talking about it more in how the… Hedvig, this is the measure of whether you became a real Australian or not. When I say D&M, do you know what I mean?
DANIEL: Oh, I know.
HEDVIG: No.
BEN: If you and your mates in Canberra got together and you had some D&Ms, do you know what I’m talking about?
HEDVIG: Dinner and…
BEN: No, sorry.
DANIEL: A movie! [LAUGHS]
HEDVIG: Memories!
BEN: It’s okay though, because who would want to be a real Australian? We’re garbage anyway. DEEP AND MEANINGFULS is what that means.
HEDVIG: Oh!
DANIEL: That’s it. D&M. Yep.
BEN: So, in Australia, we’ve sort of funly called trauma dumping deep and meaningfuls, where you sit down and you just like… you get real with someone.
HEDVIG: Oh, my god, my mom loves that.
BEN: And that’s what I’m seeing TRAUMA DUMP get used as in TikTok now, where people will very much clearly be like, “Oh, yeah, and I was with my mates and just like, I just trauma dumped.” But it’s in a more positive sense than everyone just being distressed at the end of it. That make sense?
HEDVIG: Yeah.
DANIEL: We’re letting ourselves hang out a little bit.
BEN: Authenticity and vulnerability is how I am seeing TRAUMA DUMP used a little bit more now.
DANIEL: Those are two really good things.
BEN: Yeah! But also, awful and terrifying. So, you know, there’s that too.
HEDVIG: I mean, one thing that helps with some of these things is if you tell people beforehand. Like, I’ll tell Ste, “In five minutes, I would like to talk to you about what we’re going to do today and make a list and it’s going to be a bit mentally taxing.” You can prepare a thing. You can be like, “I think I’m going to trauma dump.” [CHUCKLES] That actually makes things a little bit easier.
DANIEL: You’re a good partner. Way to give him a heads-up.
BEN: Go you, man. Go you.
DANIEL: So KANEKALON, DELULU. Sorry, there was one that came up and I didn’t write it down. What did we…
HEDVIG: RAT GIRL SUMMER!
DANIEL: Oh, yeah.
BEN: [LAUGHS] No notes necessary because Hedvig is square within her own Rat Girl Summer.
HEDVIG: Yeah!
DANIEL: DELULU, RAT GIRL SUMMER, BLUE TICK and derivatives, SKEET and TRAUMA DUMP: our Words of the Week. Here’s a comment from Wolf via email. hello@becauselanguage.com. Hey, Hedvig. Remember when you and I were talking about “glass” terms like the glass cliff and the glass ceiling? Glass walls.
HEDVIG: Glass floor, I think, as well.
DANIEL: There was that. And we wondered what to call it, when usually women, take on a kind of pastoral role in the workplace, doing the work of helping everybody sort out their emotional stuff on top of your regular work. What do we call that thing? We couldn’t think of a good glass term. We thought of GLASS PILLOW, GLASS YOKE.
HEDVIG: GLASS PASTORAL CARE?
[CHUCKLES]
DANIEL: [STRAINED PAUSE] Thank you. That’s so good. But you know, Wolf’s got one. Wolf says…
HEDVIG: [LAUGHING HYSTERICALLY]
DANIEL: Staying positive, it just smooths the way, just makes the show easier.
BEN: What were we saying about authenticity just before? I can’t remember.
[LAUGHTER]
DANIEL: I didn’t say I was good at it!
BEN: [LAUGHS]
HEDVIG: Love it, love it. Yeah, what’s up?
DANIEL: Wolf says, “GLASS MILLSTONE. You were so nearly there,” says Wolf. A millstone. You know what those things are.
HEDVIG: It’s something that grinds.
DANIEL: Yeah. It’s a gigantic stone wheel and a donkey turns it and it grinds up grain. But because Jesus allegedly uses it in the New Testament, it’s become known as a very unpleasant problem or responsibility that you cannot escape from.
BEN: Oh, yeah. Like you’re the donkey. Yeah, okay, I get it.
DANIEL: Well, the allusion that Jesus made was: if anybody hurts a child, it’s better that a millstone was tied around their neck and thrown into the sea. That’s the allusion there. So, you can’t get rid of this millstone, it’s around your neck. Glass millstone?
HEDVIG: I don’t like it. I think it requires a lot of biblical knowledge to understand it, which maybe you and Wolf have.
DANIEL: Feh on that.
BEN: Feh, feh, feh.
DANIEL: I say.
HEDVIG: Sorry, Wolf. I think it’s going to work well in Utah.
DANIEL: We’re still looking. We’re still looking.
BEN: [LAUGHS] That is the best phrase I’ve ever heard for like, “I don’t care for this evangelical shit. I think it’ll be big in Utah.” [LAUGHS] I’m going to use that. That’s great.
DANIEL: Thanks to Dr Emily Bender and to Dr Jack Hessel. Thanks to everyone who gave us ideas for the show. Thanks to the team from SpeechDocs who transcribes all the words. Dustin of Sandman Stories Presents, who keeps repping us to everyone. And most of all, our patrons who support the show and keep us going. Thanks, all.
BEN: Speaking of supporting the show, if you would like to do that, there’s several ways you could do so. The first, and I reckon best and coolest, is just tell some people about our show. If you’re listening to this and you’re like, “Damn, this show’s wicked. I like it,” tell some people. Because that’s what I do all the time, when people are like, “Oh, yeah, I was a bit bored the other night. And then, I put on, like…” someone give me a name of a really bad reality TV show because I don’t know any off the top of my head.
HEDVIG: Real Housewives of North Sydney.
BEN: “I was really bored last night and tired, so I just put on Real Housewives” and I’m just like, “Stop that shit immediately! Listen to some podcasts. They’re still fun, they’re still engaging, but they’re not brain cancer the way reality TV is.” And you do two things by doing that. You help us get to more ears, which is really cool for us. But you also save a person from garbage television. So, really, who’s the hero in that situation? You are. If you recommend us to people.
HEDVIG: Ben.
BEN: Yeah?
HEDVIG: If there was the scale of 100% ketchup versus 100% vegetables, and reality shows are 100% ketchup and BBC News is 100% vegetables…
BEN: Well, hang on. Well, we’re doing different things here though, because reality TV isn’t trying to be like a fourth estate function. So, from reality TV to Chernobyl, the drama show.
HEDVIG: No, no, no. This is category of things you put on when your brain needs a little bit of a rest.
BEN: Oh-kay.
HEDVIG: Maybe BBC… Oh, uh, maybe it is.
BEN: Okay.
HEDVIG: If zero is BBC and 100 is Real Housewives of North Shore, where do we fall? Because I don’t think we fall on zero.
BEN: No, absolutely not. No, no, no. We can definitely be, like, lean-back media for sure. I’d say somewhere around the middle, to be honest.
DANIEL: I think so too.
BEN: We get the swears on. We have some laughs. We talk about some important things, but not like crazy deep important things? So, we’re a little bit in the middle.
HEDVIG: Half vegetable, half ketchup.
BEN: Yeah. We are broccoli with ketchup on it. Bleugh. [LAUGHS] God. What a terrible mental image. But you know what? If you don’t want to recommend us to a friend, or if you’re like me and you don’t really have any friends, there’s other ways that you can support us. You can give us feedback on the places where our podcast pops up, like Apple Podcasts or Stitcher or any of those places. You can… and this is really fun, you can leave us a voice message on SpeakPipe about what you liked about the show so that we can put it in our show and we can hear your voice in our show and it just sounds cool and wicked and fun. And you can also send us an email, hello@becauselanguage.com is how you can do that. But then, there is also one more way that you can support us. I think Hedvig might have something to say about that.
HEDVIG: If you’re a big fan of our Ketchup Broccoli Show…
BEN: [LAUGHS] Oh, god. I’m sorry, everyone!
HEDVIG: …one of the ways you can help us is to become a Patreon. A supporter on Patreon. And this helps us do a lot of things. It involves money, it does. And we use that money for good things. We pay the bills for various expenses we have. We use it to transcribe our shows so that you can read them and search them so if you want to hear where we said Ketchup Broccoli, you can find this show.
DANIEL: Here’s one.
HEDVIG: Oh?
DANIEL: When Daniel is agreeing with someone, is he more likely to say PRECISELY or EXACTLY? Now, you can look that up.
BEN: [LAUGHS]
HEDVIG: I think PRECISELY is my guess. And you can support us at different levels. You can get bonus episodes, mailouts, shoutouts. There are different benefits to different levels, but there are benefits even at the very lowest of levels. So, get on and join the team. All the patrons, for example, regardless of level, get invited to our live shows and hang out with us on our Discord. And we’re going to give a special shoutout to the people who qualify as top patrons. And they are Iztin, Termy, Elías, Matt, Whitney, Helen, Jack, PharaohKatt, LordMortis, gramaryen, Larry, Kristofer, Andy, James, Nigel, Meredith, Kate, Nasrin, Joanna, Keith, Ayesha, Steele, Margareth, Manú, Rodger, Rhian, Colleen, Ignacio, Sonic Snejhog, Kevin, Jeff, Andy from Logophilius, Stan, Kathy, Rach, Cheyenne, Felicity, Amir, Canny Archer, O Tim, Alyssa, Chris, Laurie, aengry balls, and Tadhg. And our newest patrons on the Listener level are Ned and Sam, who is a pal from from the radio on the Talk the Talk days, and Holly, Spider Hunter of Taipei, which I think sounds really cool. And Katrina bumped her pledge up to the Listener level. Thank you to all of our generous patrons.
DANIEL: Got a couple of late entries. Nikoli bumped up to the Supporter level. Thanks. And Mitch becoming a patron at the friend level. Thanks, folks. The music you are hearing right now was composed and performed by Drew Krapljanov of Didion’s Bible and Ryan Beno, both great listens. Thanks for listening. We’ll catch you next time. Because Language.
Apparently, Dax from SpeechDocs is not the same as the actor, Dax Shepard.
BEN: Um, that is not surprising to me. [CHUCKLES] That is not a revelation.
DANIEL: Yeah, but there’s always a chance, you know? I don’t know. Just…
[Transcript provided by SpeechDocs Podcast Transcription]